1
Content guide
Public transportation travel benefits urban systems in many ways, such as reducing traffic congestion, reducing air pollution, and saving energy. The built environment of a city and the cost of travel time are considered to be the two key categories of factors that influence people's travel preferences and behavior during transportation trips. Therefore, understanding people's feelings and perceptions about the spatial and temporal characteristics of public transportation travel process can not only improve the supply and operation of public transportation, but also encourage more people to use green and sustainable travel modes and increase travel well-being.
In recent years, neuroimaging methods, led by functional magnetic resonance (fMRI), have been increasingly applied to urban science (e.g., urban well-being, landscape assessment, and navigation, among a range of other topics) to capture the connections between the human brain and urban perception and behavior. In the field of transportation geography, despite calls to integrate human travel perceptions with environmental psychology and cognitive science, mainstream research still follows traditional behavioral survey methods, making it difficult to provide comprehensive and objective measurements of the complex spatio-temporal experiences of travel.
Therefore, in order to provide neuroimaging evidence of human spatial and temporal perception of public transportation trips, nine subjects were recruited to conduct a 3-week traffic trip experiment in a real environment (Beijing city) and returned to the laboratory to undergo fMRI scans. During the scanning period subjects carefully recalled their public transportation trips. Using representational similarity analysis (RSA), this study revealed for the first time a quantitative relationship between the spatial and temporal characteristics of public transportation trips and brain activity patterns.
2
Research Content
In this study, to ensure high ecological validity of the results, an empirical study of public transportation trips was conducted in a real environment and fMRI scans were returned to the laboratory at the end of the trip to recall the feelings during the trip. Similar to the number of subjects in other life-record type fMRI high-level studies, this study recruited nine subjects (four males, five females, mean age 21.7 years; number of subjects) who agreed to participate in the public transportation travel experiment and to record their travel trajectories and logs. In addition, they had no history of neurological disorders and were able to participate in a recall experiment based on fMRI scans.
In the public transport travel experiment: subjects were asked to choose public transport for each day based on a predetermined travel destination and to take pictures of key landmarks during each trip as 'anchor points' for subsequent recall experiments. The subjects were asked to record track data using a cell phone app during each trip, and to fill out a questionnaire about the trip to collect their behavioral data after completing the trip. After a week of each trip, subjects were asked to review the landmarks they had photographed and the questionnaires they had filled out to reinforce their memory of the trip. After completing all public transportation trips, subjects verbally described their subjective perceptions of all trips (Figure 1).
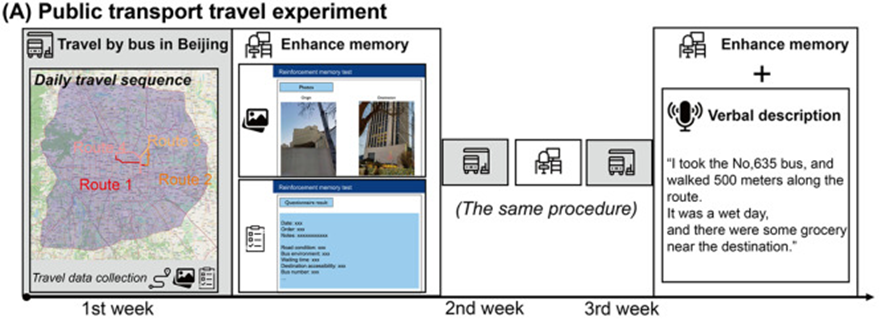
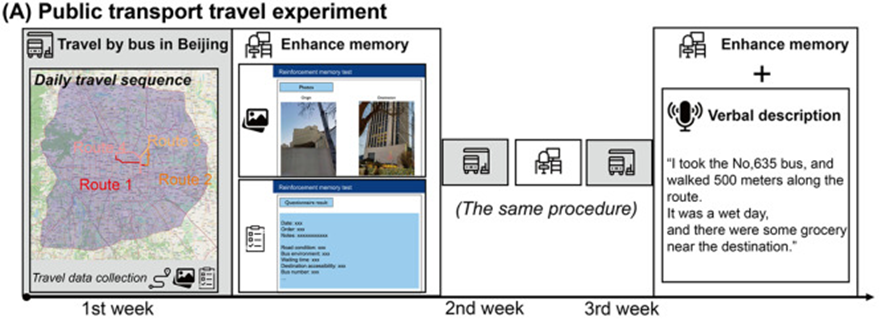
Figure 1 Public transportation travel experiment
In the fMRI scan-based recall experiment: the entire recall process was done in the MRI lab. fMRI experiments were designed using an event-related approach, with each trip-related 'anchor' presented for 10 seconds, during which subjects viewed photographs of landmarks taken at the start and end points of the trip, and based on which they They then had 3.5 seconds to key feedback on the level of detail they recalled about the trip (remembering more detail/remembering less detail), and all data remembering less detail were excluded for subsequent analysis. Then after a randomized 4-12 second rest process, subjects proceeded to recall the next trip (Figure 2).
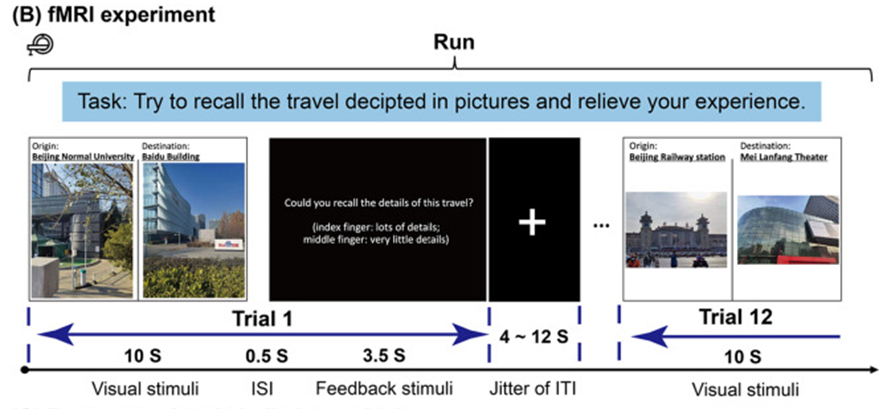
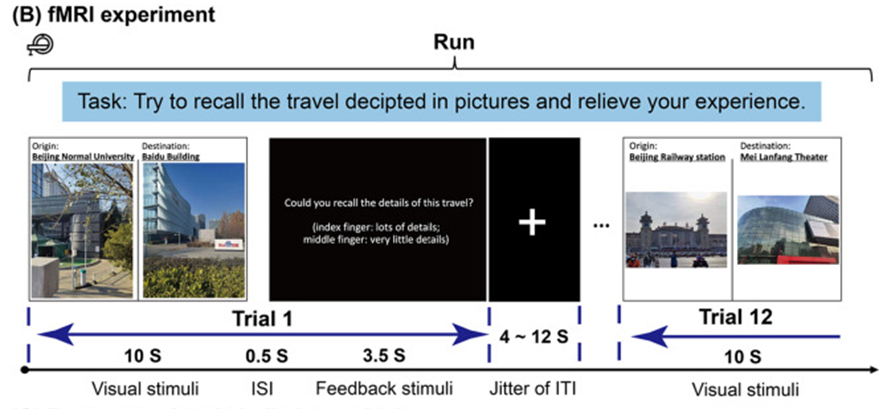
Figure 2 Recall experiment based on fMRI scan
The core method in this study is the classical representational similarity analysis (RSA) approach in cognitive neuroscience for assessing the relationship between spatiotemporal features and brain activation patterns. The method does not directly assess the relationship between spatiotemporal features and brain activation, but rather assesses the correlation between spatiotemporal feature differences and brain activation differences (Figure 3). In this study it can be specifically divided into the following three steps. First, the first step entails estimating the brain activation pattern Bap (i.e., a three-dimensional array composed of the beta values of all brain voxels at the time of recalling that trip) for each public transportation trip.


In the present study, we were concerned only with the activation patterns of core brain regions associated with spatiotemporal perception and situational memory, specifically the bilateral hippocampus, the parahippocampal region, and the postsplenial complex region. The activation patterns of these core regions are a subset of the brain, denoted as.


Based on the activation patterns of these core brain regions for travel perception, we can construct a similarity matrix (RDM) of representations within the brain. The matrix is diagonally symmetric and each cell in the matrix represents the "distance" between the activation patterns of two different travel perceptions in the brain. In this study, the "distance" between activation patterns is measured by Euclidean distance.


Second, the second step requires quantifying the spatio-temporal features of the travel routes and constructing a spatio-temporal feature dissimilarity matrix (STCDM) with the same dimensions and dimensions as the RDM. A total of 14 spatial features (Euclidean distance, route distance, curvature, number of turns, number of intersections, number of unique streets, deflection angle of the route from a right angle, deflection angle of the route from the destination, azimuth, degree centrality, proximity centrality, slope, number of POIs, and land use type) and 8 temporal features (travel time, waiting time, in-vehicle time, out-of-vehicle time, travel date, travel order, number of interchanges, and travel speed). These route-based spatio-temporal features were calculated based on the log and trajectory data of the subjects' public transportation trips and combined with open-source GIS data (including Beijing's road network data, POI data, digital elevation model data, and land use type data). See the original article for the specific index meaning and reference. Then, we obtained the "distance" between the spatio-temporal characteristics of two pairs of routes by simply making a difference. Such distances are compared with brain representation distances in the same dimension.
The final step is to compare two matrices of the same dimensions using a nonparametric permutation test to determine which features are closely and significantly associated with brain representations and represent the spatio-temporal elements that we may capture when traveling for perception.
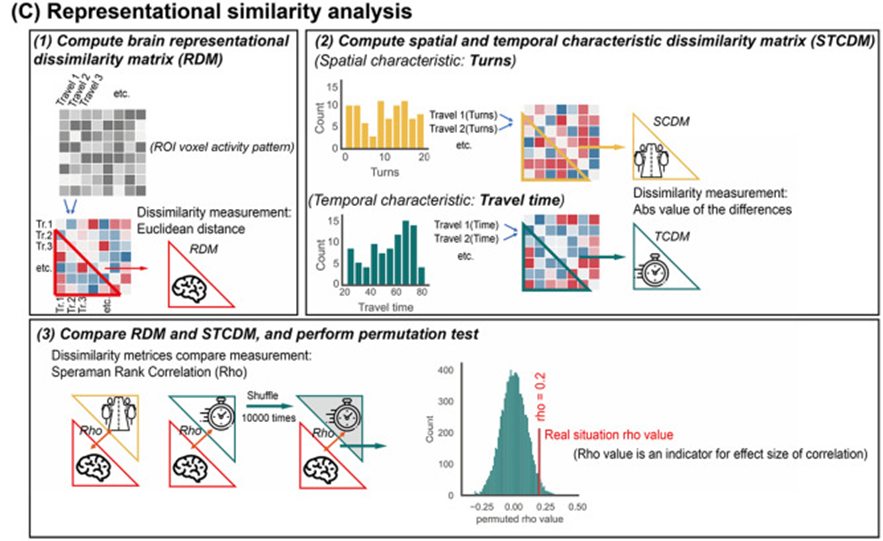
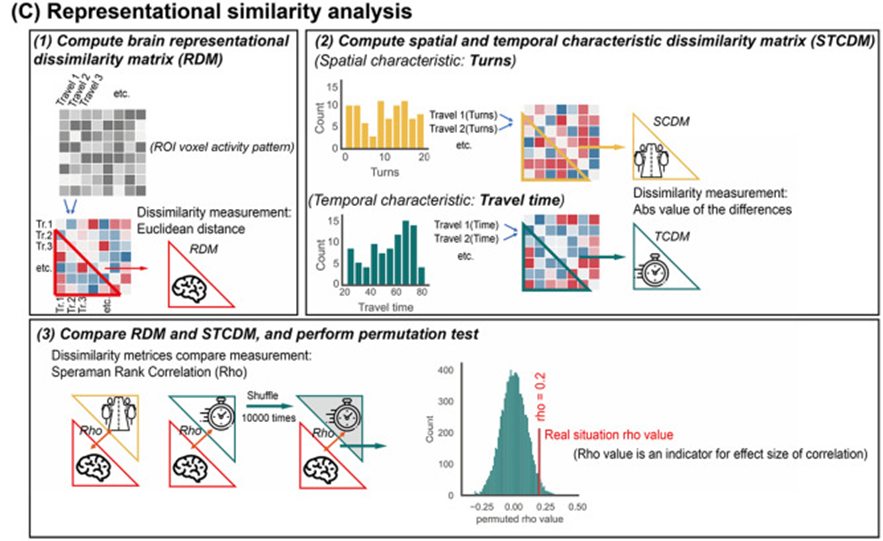
Figure 3 Characterization similarity analysis method
3
Study results
For the public transportation travel experiment, we collected a total of 651 unique travel routes (Figure 4A), and the spatio-temporal characteristics of each route were quantified and calculated, and the spatio-temporal differences between travel routes were visually reflected (Figure 4BC). In addition, weThe internal correlations between spatial and temporal features were examined (Figure 4DE). The results show that the correlation between the individual spatial features of the travel routes is high, but the correlation between the temporal features is low, which indicates the high uncertainty of public transport travel times and costs.
For the results of the RSA analysis, we first conducted statistical analyses at the individual subject level and found substantial differences in the representation of spatiotemporal features across subjects' brains (see the original supplemental material). Furthermore, although subjects' brain activity patterns may be significantly correlated with multiple spatiotemporal features simultaneously, the effect sizes of these features are not high, reflecting the fact that the perception of spatiotemporal features in real environment trips is highly complex and not the only factor perceived. These results were corroborated by the subjects' behavioral investigations (verbal descriptions). When describing their perceptions of travel routes, in addition to spatio-temporal characteristics, they also talked about special events that occurred during the trip, weather conditions, bus environment, and internal activities and emotions.
Finally, we examined the RSA results of nine subjects at the group level and finally determined that seven spatial features (route curvature, number of turns, deflection angle of the route from the right angle, deflection angle of the route from the destination, land use type, number of POIs and slope) and two temporal features (travel time and waiting time) correlated significantly higher than zero with the activity patterns of the core brain regions of interest (Figure 5), these findings suggest that the human brain does characterize the spatio-temporal features of the public transportation travel process.
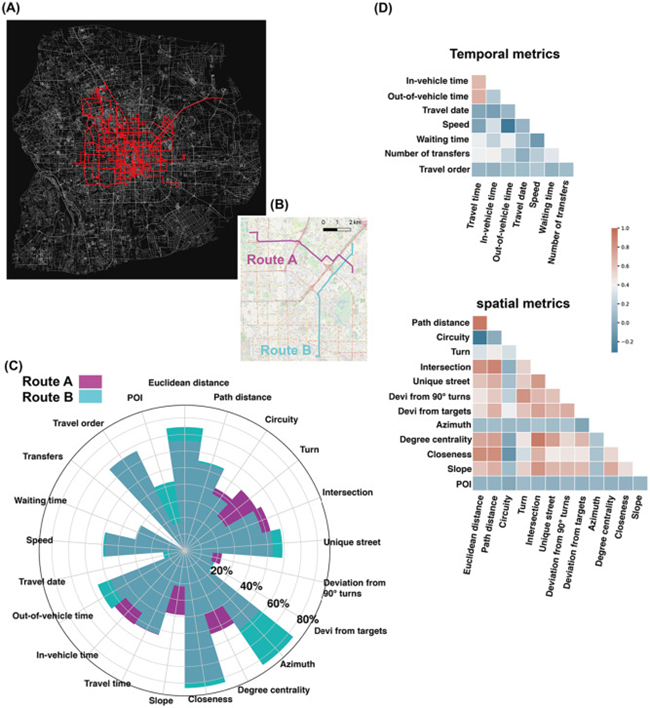
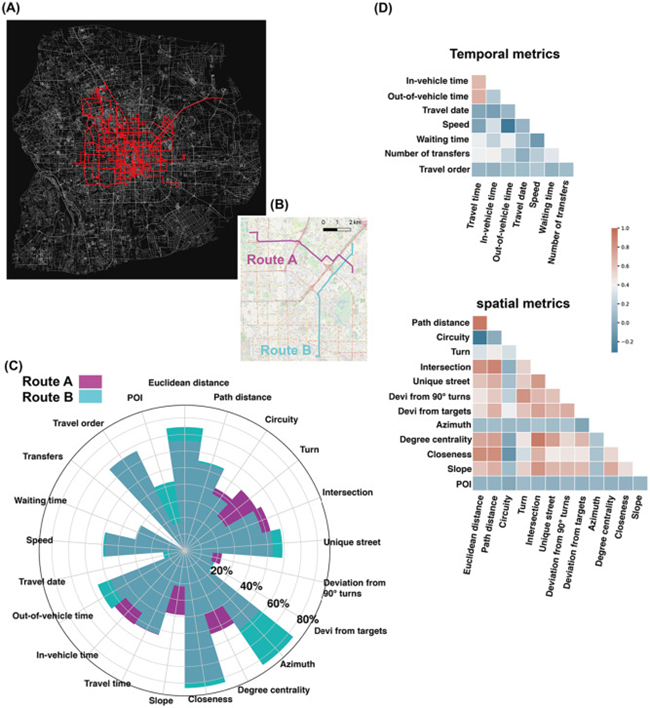
Figure 4 Experimental results of public transportation trips. (A) All travel route trajectories. (B) Two travel route cases. (C) Spatio-temporal feature values of the two travel routes; each value is normalized to the range [0,1]. (D) Spearman correlation between spatial and temporal features.
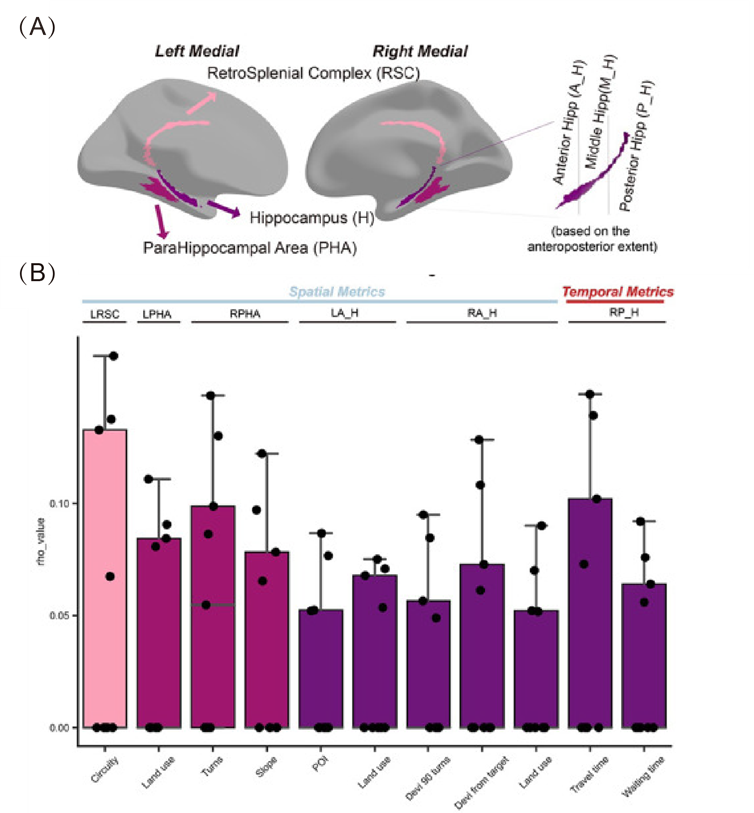
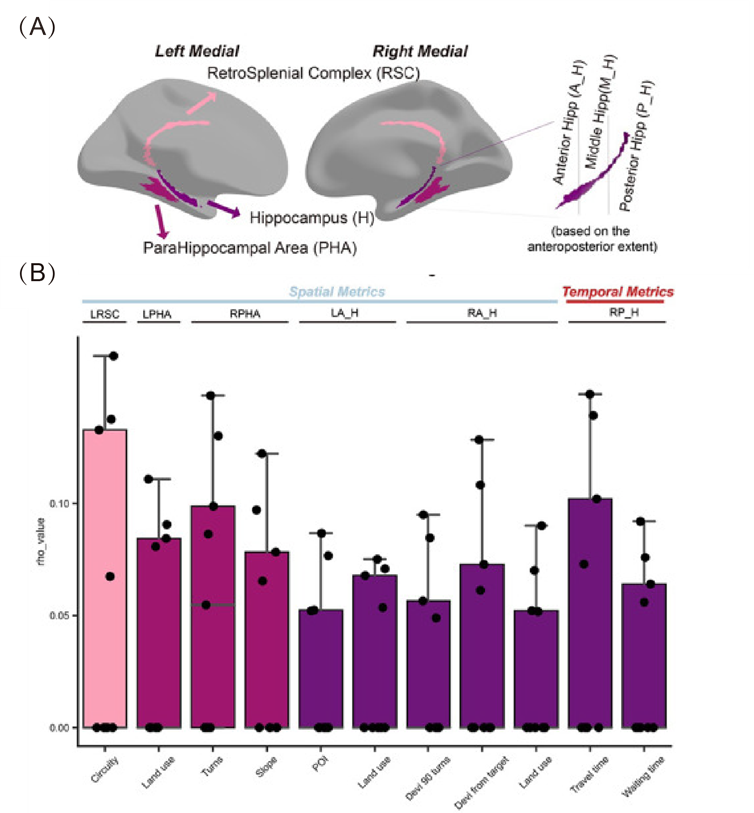
Figure 5 (A) Location of core brain regions of interest for RSA analysis in this study; (B) spatio-temporal features significantly correlated with brain activity at the group level.
4
Summary and Outlook
This study has obtained the first neuroimaging evidence on the relationship between spatio-temporal perception of public transportation trips and human brain activity through an empirical research method with the help of cognitive neuroscience, showing that spatio-temporal features such as route curvature, number of turns, route angle deviation, number of POIs, land use type and slope variation, as well as travel time and waiting time are indeed closely related to human brain activity patterns. Future research in the field of cities and transportation can use neuroimaging to better explore the causal relationships between these spatiotemporal features and traffic travel behavior, facilitating a better understanding of human activity and contributing to urban planning and public transportation from an interdisciplinary perspective.
References
Qin, T., Dong, W., & Huang, H. (2023). Perceptions of space and time of public transport travel associated with human brain activities: A case study of bus travel in Beijing. Computers. Environment and Urban Systems, 99, 101919.
DOI: 10.1016/j.compenvurbsys.2022.101919
This article comes from the WeChat public number: EVERLOYAL