1
Content guide
Target detection is the basis for understanding and analyzing remote sensing images, which is widely used in the fields of environmental monitoring, urban management and disaster assessment. And human visual attention plays a key role in remote sensing target detection. Compared with natural images, remote sensing images have a wider spatial scale and a unique top-down perspective, and at the same time, they are more susceptible to the dynamic effects of changes in the geographic environment. However, most of the current understanding of visual attention comes from natural images, and there is a lack of research on human attention in remote sensing image target detection.
In visual attention studies for natural image target detection, eye movement and electroencephalography (EEG) techniques are widely used to capture the cognitive processes of human visual attention. Existing studies have categorized human attention in target detection into four stages: guidance, allocation, selection and recognition.
From this we propose three key issues for target detection in human remote sensing imagery:
-
What image properties guide human visual attention?
-
What factors influence the allocation of attention during target search?
-
How do humans select and recognize targets?
To answer the above questions, we conducted a target detection cognitive experiment using an airplane as a target, and simultaneously collected EEG and eye tracking data from 40 remote sensing interpretation professionals. Using eye-tracking metrics and gaze-related potential (FPR) analysis, this study provides the first comprehensive and reliable understanding of human visual attention in remote sensing target detection.
2
Research Content
In this study, 1000 images were selected from the DIOR remote sensing target detection dataset, and each image contained 0 to 6 airplanes. We selected 40 graduate students with professional background in remote sensing and experience in interpretation as subjects through a questionnaire test. Each subject was asked to view 500 remote sensing images and find all the airplanes in the images within 3 seconds. Eye movement and EEG data were acquired synchronously by an SMI RED250 eye-tracker (250 Hz) and a NE Enobio 32 EEG (500 Hz, 32 channels) (Fig. 1).
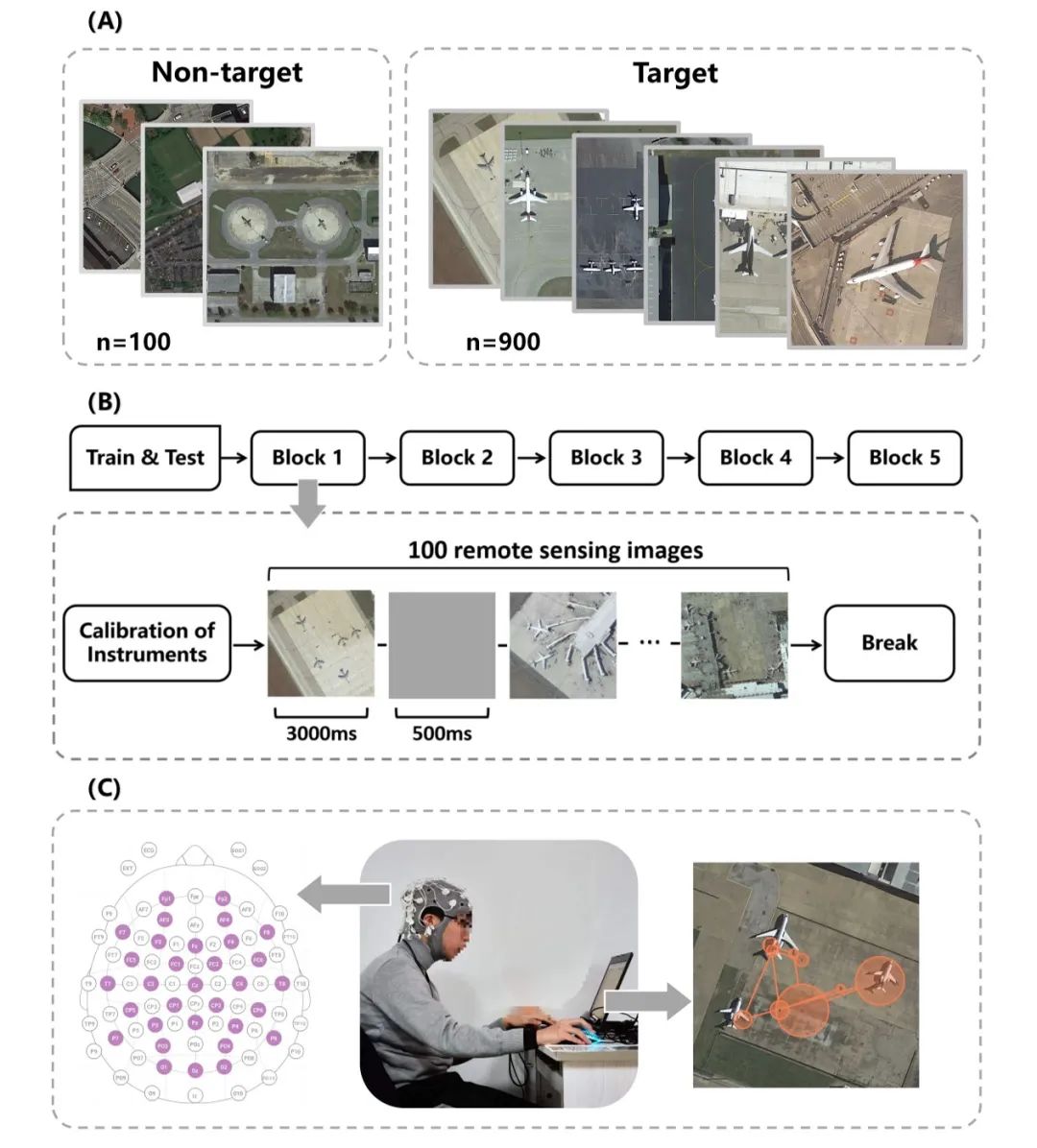
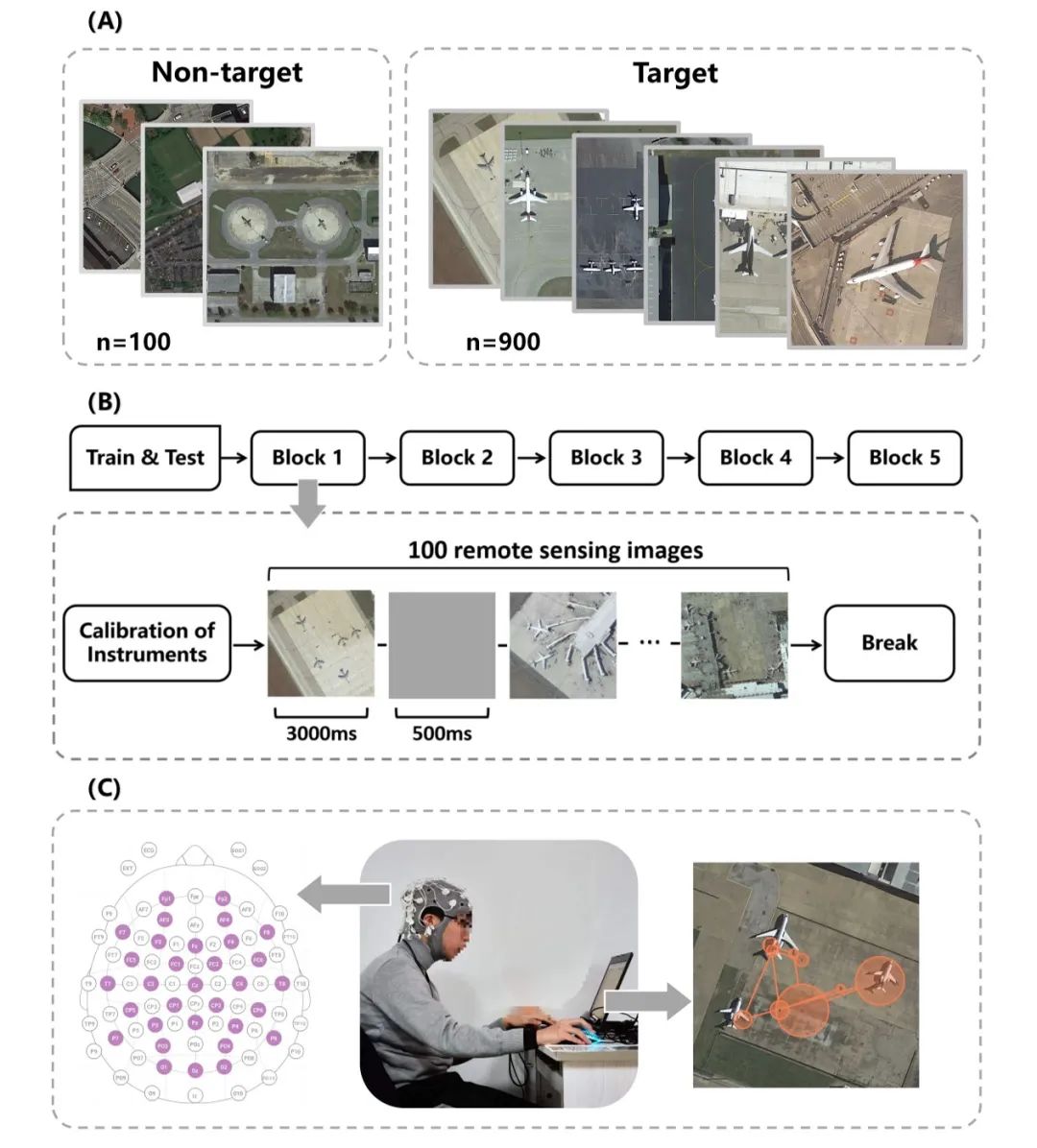
Fig. 1. Eye movement and EEG based remote sensing target detection experiment.(A) stimulus material; (B) experimental procedure; (C) data collection process, left: EEG electrode arrangement, center: photograph of the experimental scene, right: eye movement trajectory.
For the image features guiding the subjects' vision, this study divided the remote sensing image into three regions, namely, target, interferences and background, based on the eye-movement heat map and target labeling data, and statistically analyzed the hue, luminance, saturation, grayscale nonlinearity, edge density and image information entropy of each region, and performed the Mann-Whitney rank sum test.
To address the factors affecting subjects' attention allocation, the present study counted the duration of gaze, the number of times of gaze, and the average duration of gaze in different areas, designed two standardized difference indices to measure the differences in subjects' attentional behaviors to targets and distractors, and calculated the correlations between the gaze differences and the total area of the target, the average area of the target, and the number of targets.
The cognitive process of selecting and recognizing a target was analyzed using the Fixation-related potential (FPR) method. The FPR was calculated by first selecting specific gaze points as "triggering events" and extracting the data segments related to these events; then baseline correction and superimposed averaging were performed to eliminate non-specific potential changes and improve the signal-to-noise ratio; finally, the FPRs under different conditions were compared to investigate the differences in the allocation of visual attention and the processing of visual information. Differences.
3
Study results
The results of the attention index showed that the subjects seldom paid attention to background areas with high visual complexity. This selective attention not only effectively excluded the interference of complex environmental factors, but also significantly reduced the cognitive load on the visual system. (Fig. 2 C) Further comparison of image features showed that hue and luminance were the key factors dominating subjects' visual attention allocation. Notably, saturation in remotely sensed images did not significantly affect subjects' attention allocation compared to ordinary images. In addition, texture and shape features did not play a significant role in directing visual attention (Fig. 2 B).
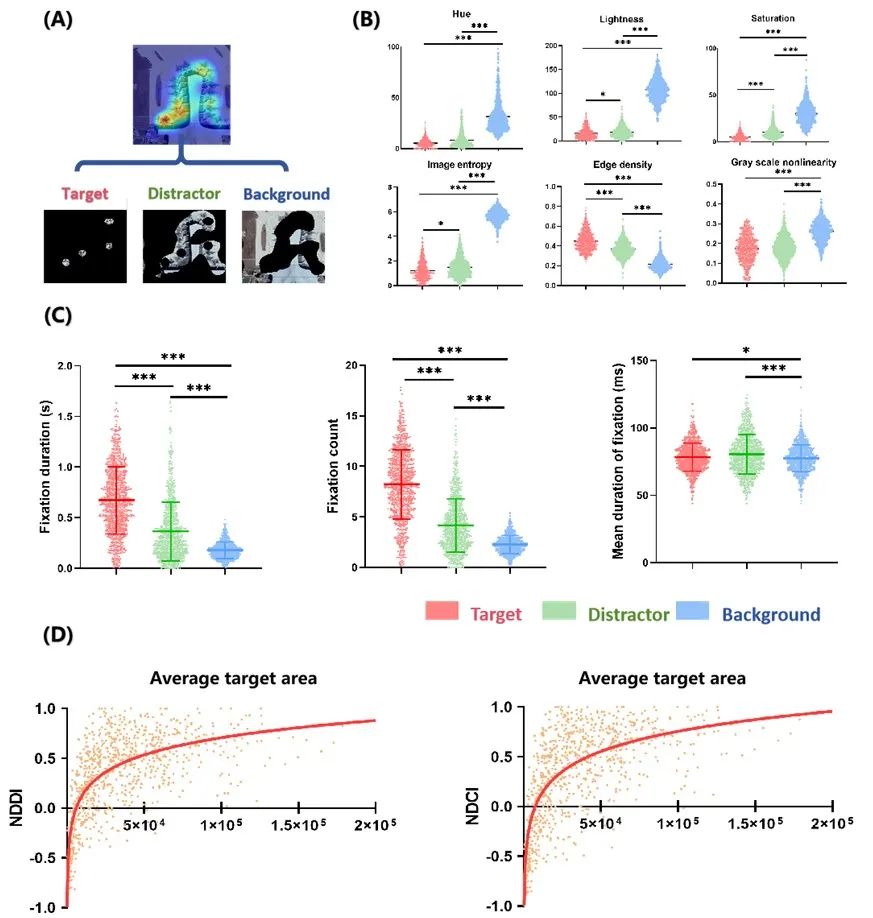
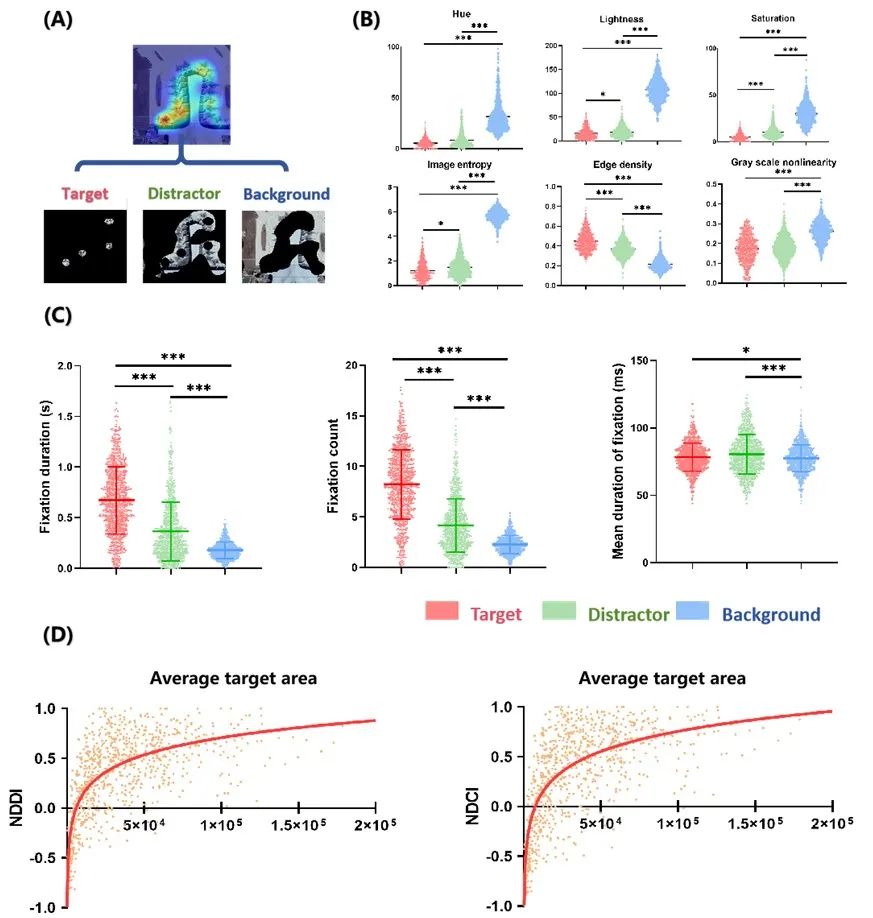
Figure 2. eye movement analysis.(A) Examples of target, distractor, and background delineation; (B) statistical results of image features of target, distractor, and background areas; (C) analysis of attentional disparity metrics for target, distractor, and background; and (D) regression analysis of the attention disparity metrics with the mean area of the target.
The results for gaze duration and number of gazes indicate that subjects allocated more attention on the target for recognizing and remembering the target. (Fig. 2 C) Unlike the findings for the natural images, as when the average area of the target decreased, subjects began to shift their attention to distractors. When the target mean area was lower than 3.91 TP3T of the whole image, subjects focused more attention on distractors. (Fig. 2 D) This shift in attention may have resulted in subjects not being able to detect all targets as quickly in remote sensing images with smaller targets.
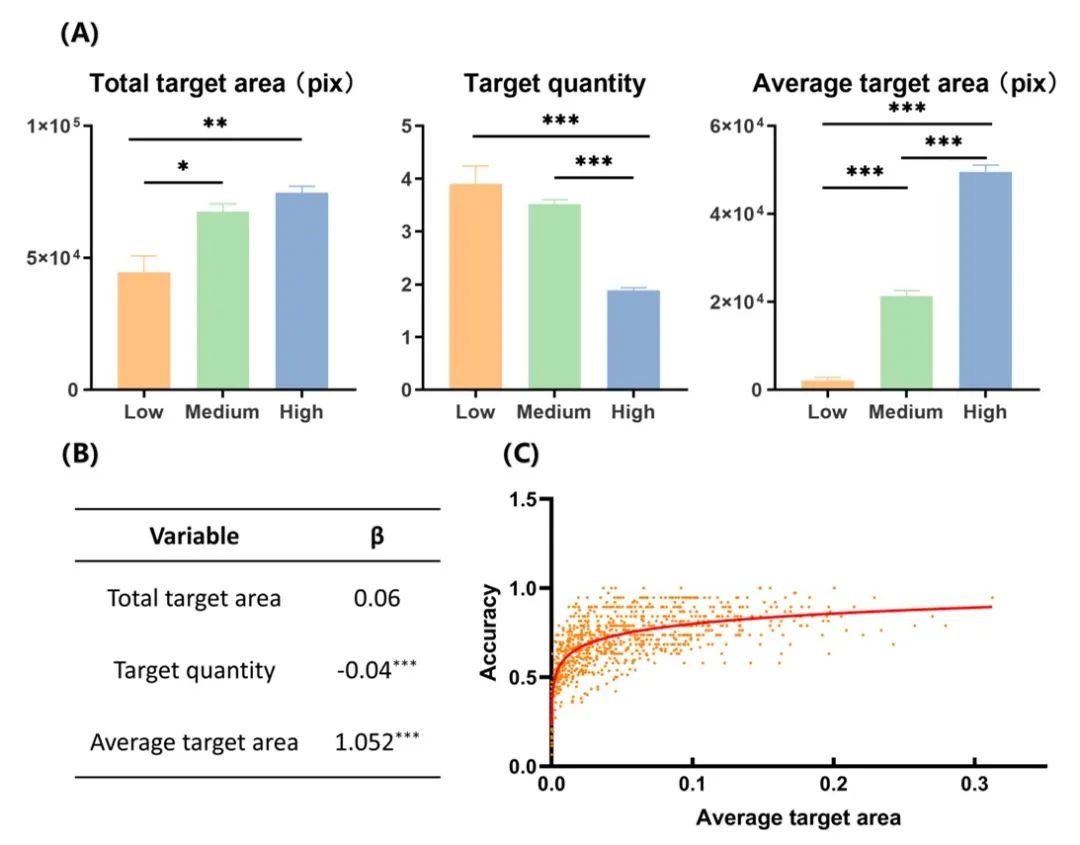
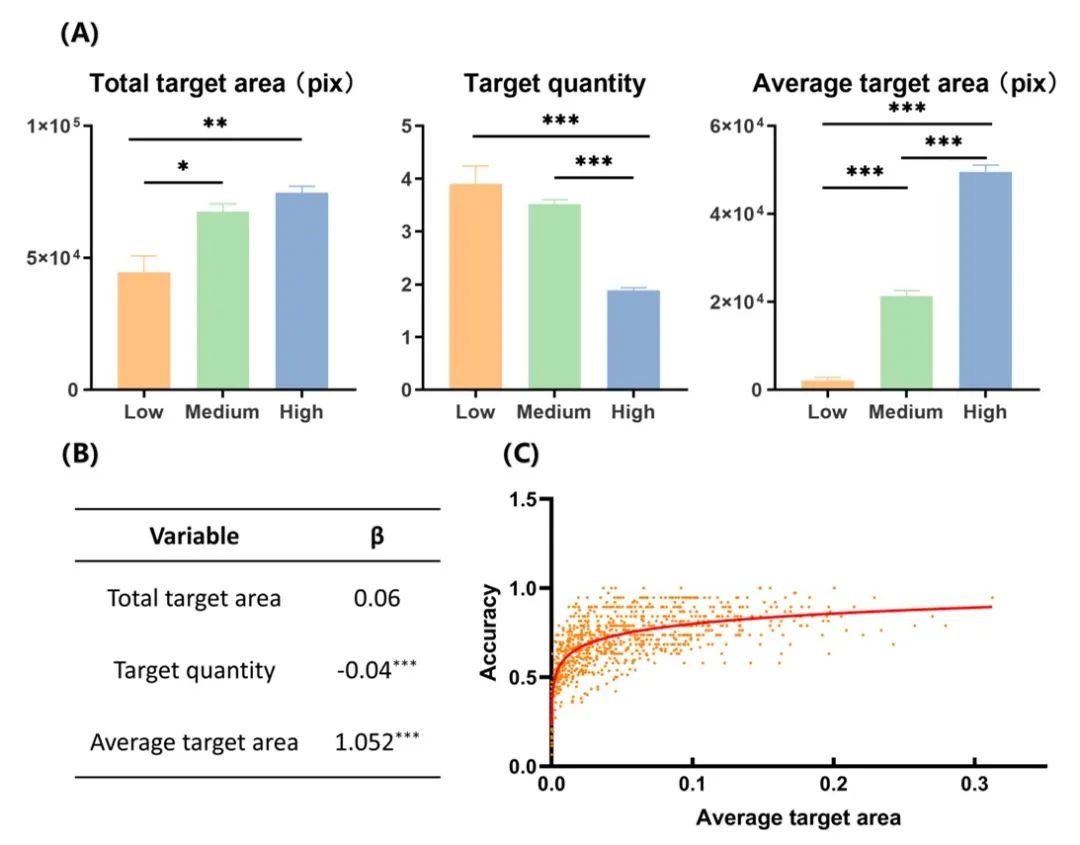
Figure 3. Correlation results between target and detection rate.(A) Differences in total target area, number of targets, and mean target area between groups with different detection rates (*: p
FRP in occipital brain regions indicated that the brain performed the same primary visual processing for both targets and distractors. (Fig. 4 B) Whereas cognitive differences between the two appeared mainly in frontal and temporal brain regions. The frontal lobe controlled the transmission and memory of primary visual letters (Fig. 4 C), and visual features were memorized, synthesized, and recognized in the temporal lobe for the target (Fig. 4 D).
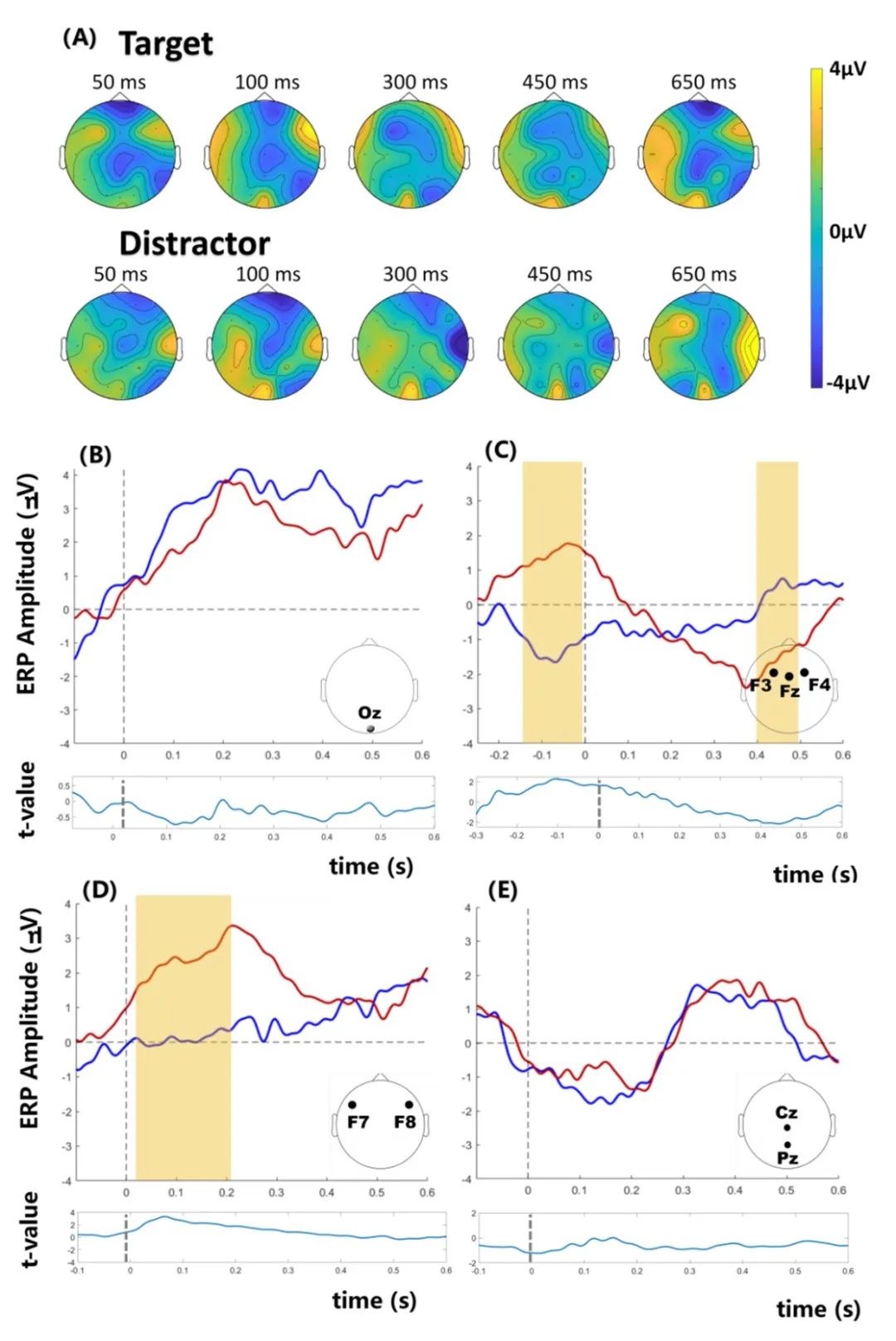
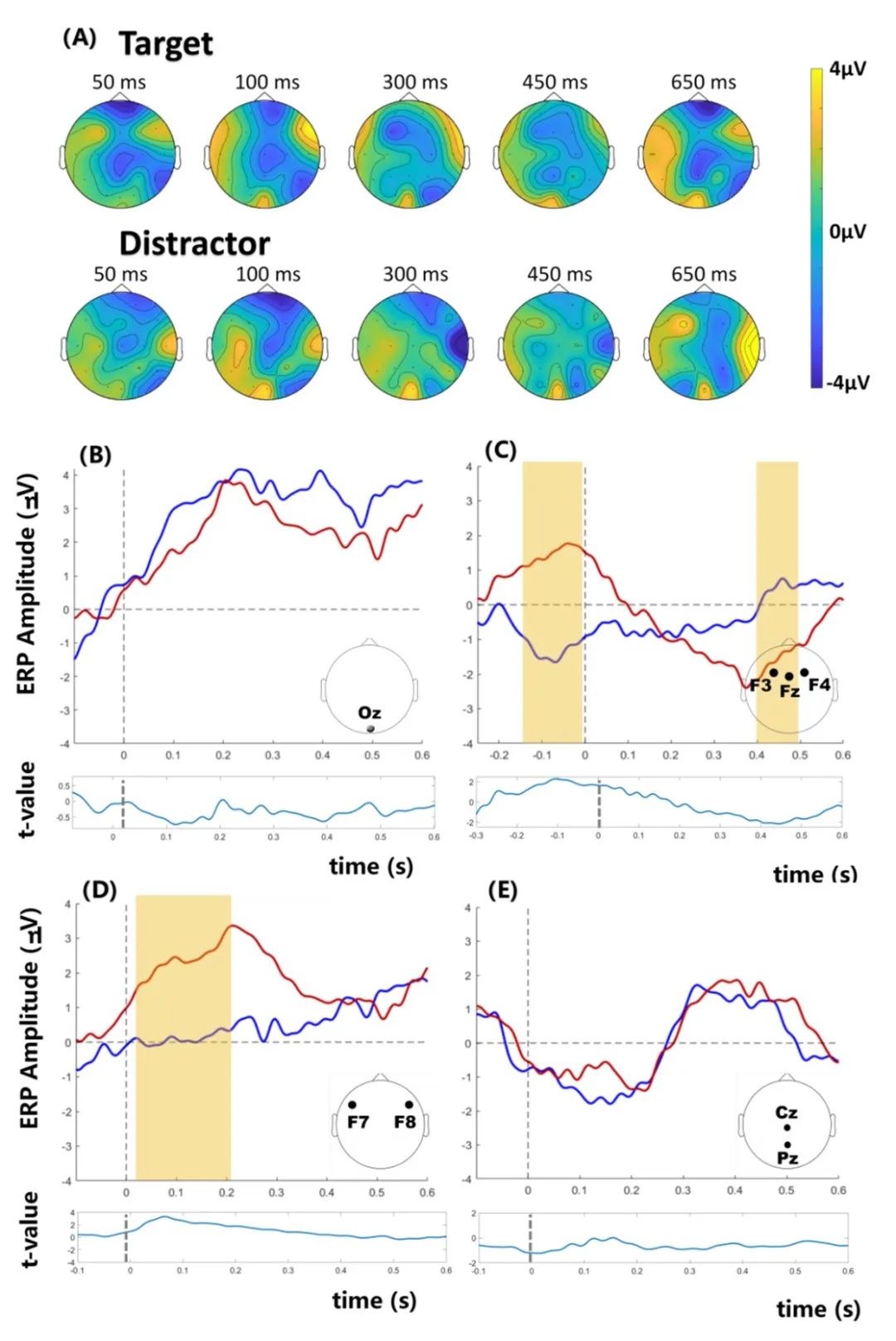
Figure 4. FRPs of targets and distractors.(A) Whole-brain topography of targets and distractors; (B-E) FRPs of targets and distractors: red lines indicate targets, blue lines indicate distractors, and shaded areas are clustering times when FRP differences are significant.
4
Summary and Outlook
In this study, joint eye-tracking and EEG explored human visual behaviors and neural responses during remote sensing target detection, revealing the cognitive processes and laws of visual attention to remote sensing images. Not only did we find the unique visual features that guide human attention in visual behavior and reveal that subjects' attention allocation depended on target size, but we also demonstrated at the level of brain neural activity that memory and processing are the key to target recognition. This study provides visual and neural evidence for understanding the human remote sensing target detection process, a theoretical basis for developing human-computer fusion remote sensing interpretation applications, and a reference and guidance for the development of intelligent detection algorithms for remote sensing.
References
Bing He, Tong Qin, Bowen Shi & Weihua Dong (2024) How do human detect targets of remote sensing images with visual attention?, International Journal of Applied Earth Observation and Geoinformation, DOI: 10.1016/j.jag.2024.104044.
Source: S³-Lab
Content layout: Wang Zhiyang
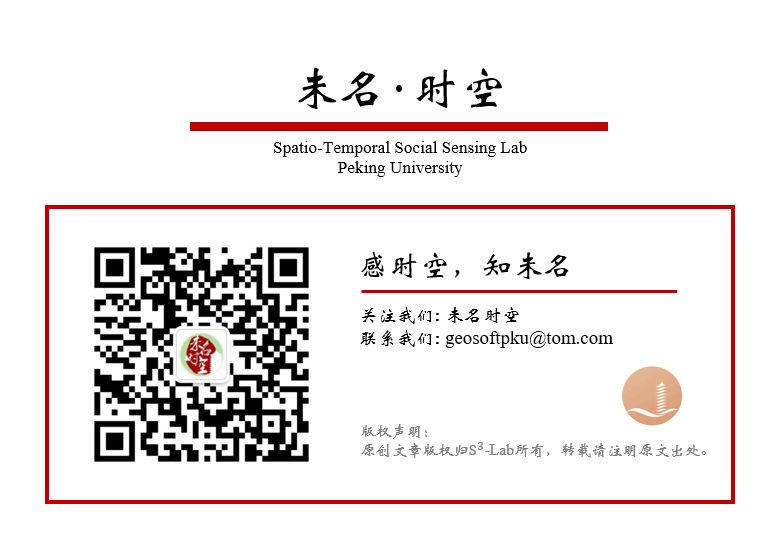
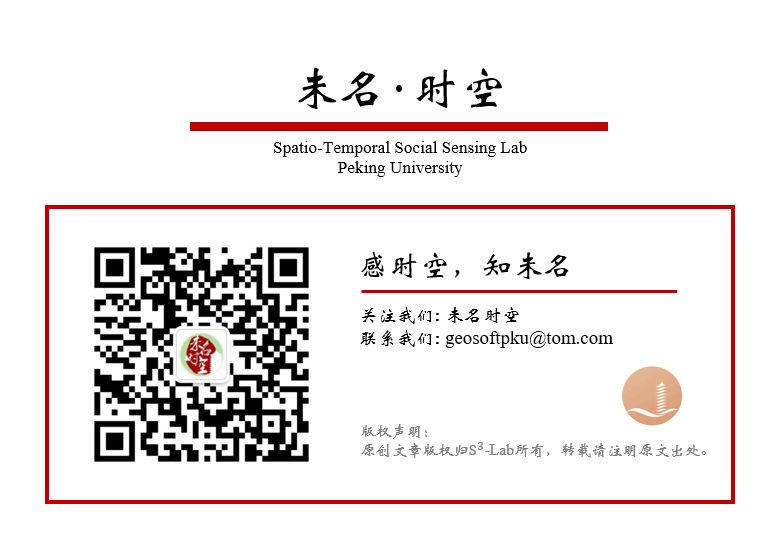