introductory
Electroencephalography (EEG) is a diagnostic technique used for brain electrical activity. It is widely used for data analysis, evaluates time and frequency sequences by measuring voltage fluctuations generated by ionic currents in neurons.EEG captures changes in synaptic transmission; when an action potential reaches an axon terminal, neurotransmitters are released, leading to excitatory or inhibitory postsynaptic graded potentials in postsynaptic membrane cells. These potentials generate ionic currents that produce local field potentials. Synchronized activity between characteristic pyramidal neurons in the cerebral cortex can be detected as an electric field. The steady direction of these neuronal currents can be prevented from canceling out, resulting in a significantly stronger overall electric field. This summation process facilitates EEG measurements, which exhibit nonlinear and nonsmooth properties. For prolonged EEG recordings, visual inspection becomes time-consuming and can be inaccurate due to artifacts.
To overcome the challenges and obtain faster and more accurate results, computer-aided techniques are utilized to process and analyze brain EEG signals.EEG signal processing and analysis typically involves four basic steps:
1) The raw signal is pre-processed, in which techniques such as filtering are used to improve signal quality;
2) Extract key features from the preprocessed signal;
3) Select methods to optimize these features and enhance them;
4) Use machine learning models or statistical tests to diagnose or identify various functional brain states.
The main objective of this paper is to explore the large number of EEG-based research applications that exist in the existing literature. The following figure shows an overview of the contents of this paper.
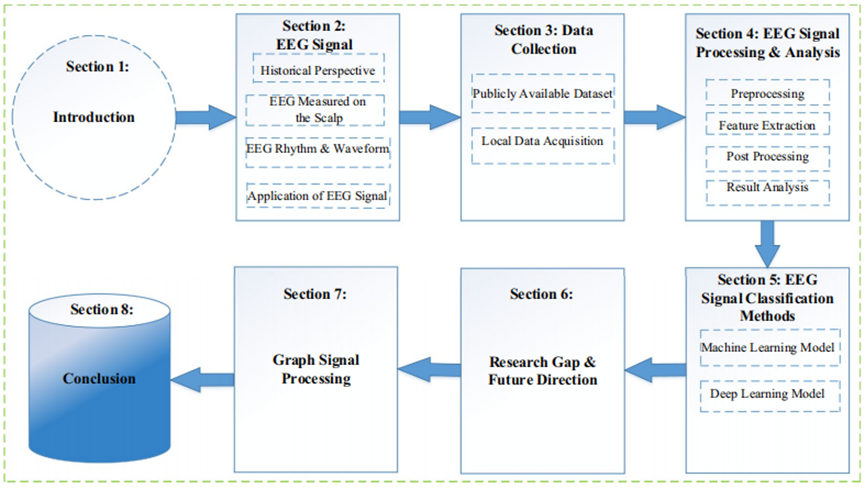
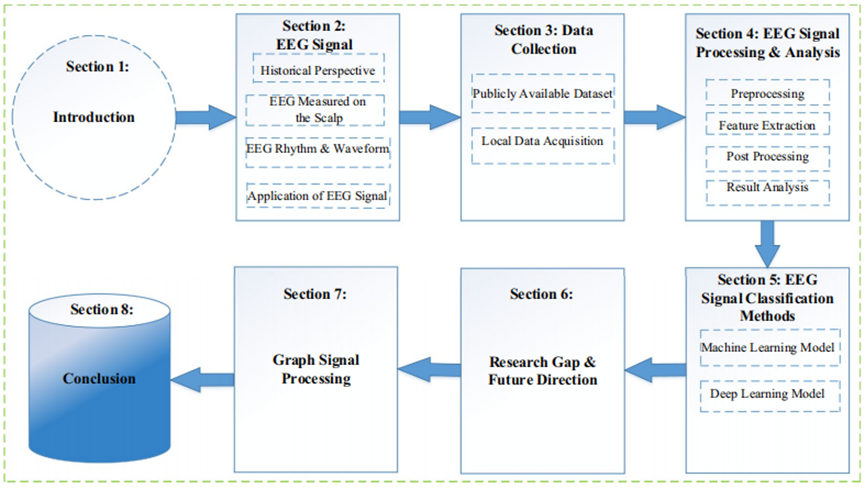
01
EEG signal








human brain
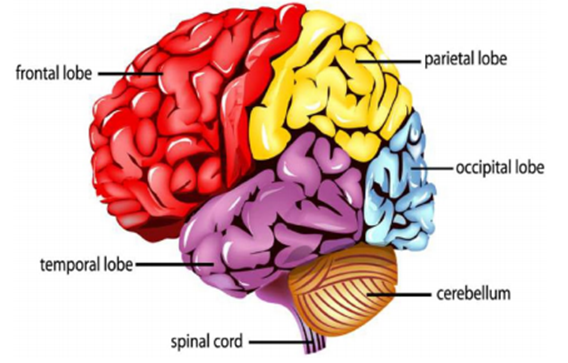
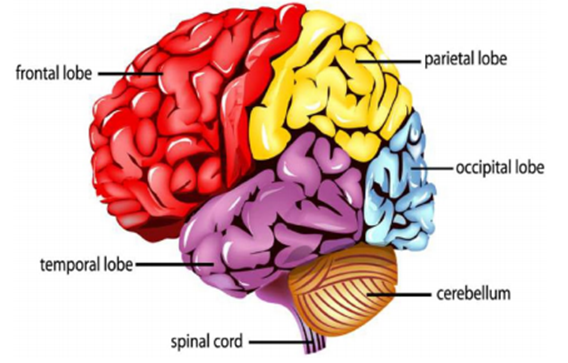








EEG rhythms and waveforms
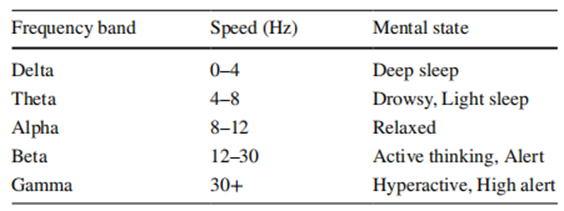
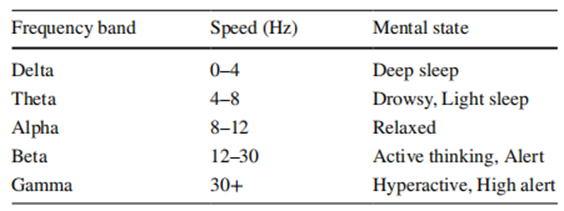








Different applications of EEG signals
02
Data Acquisition Research
03
EEG signal processing and analysis
EEG signal analysis involves four stages: preprocessing, feature extraction, post-processing and result analysis.








preprocessing
It can be divided into three steps: downsampling, artifact removal and feature scaling. Among them, artifact removal, mainly removes artifacts due to experimental settings, environmental noise, physiological signals, etc. As for feature scaling, one of the most commonly used methods is normalization, the objective function of some machine learning algorithms will not work without normalization because the range of values in the raw data fluctuates greatly.








feature extraction
Feature extraction involves extracting features from the primary signal to obtain a consistent classification, which is useful for classifying neurological disorders and other monitoring applications that use EEG signals. The most common methods such as Wavelet Transform (WT), Power Spectral Density (PSD), Statistical, Short Time Fourier Transform (STFT), Wavelet Entropy (WE), Differential Entropy (DE), Empirical Modal Decomposition, etc. The following table gives the different feature extraction techniques for EEG
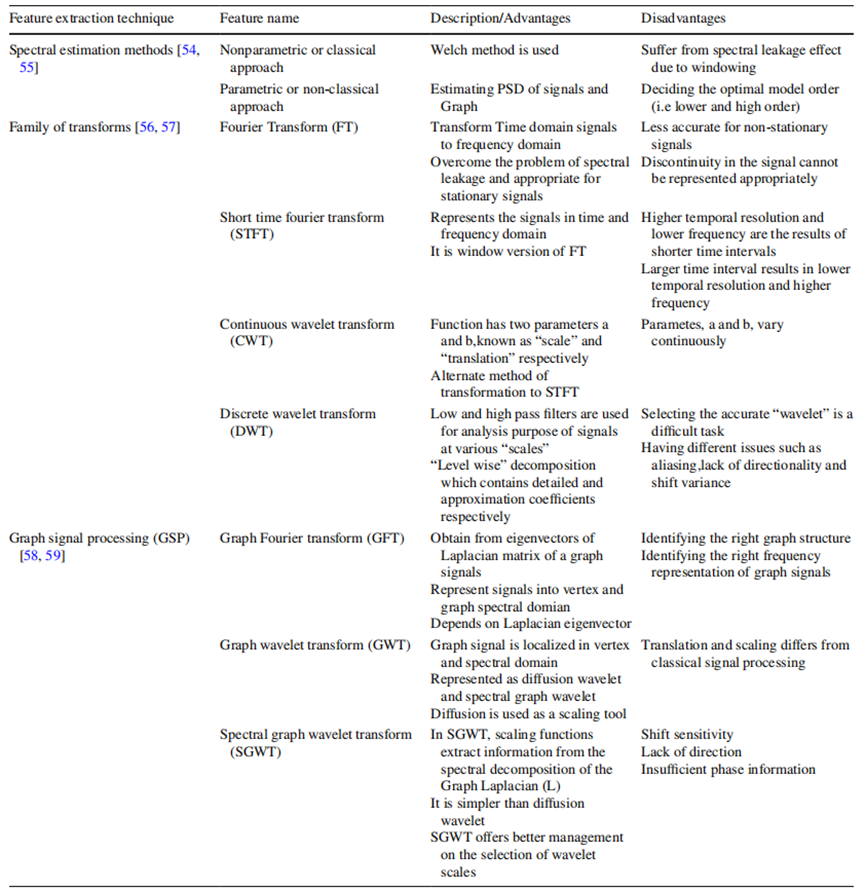
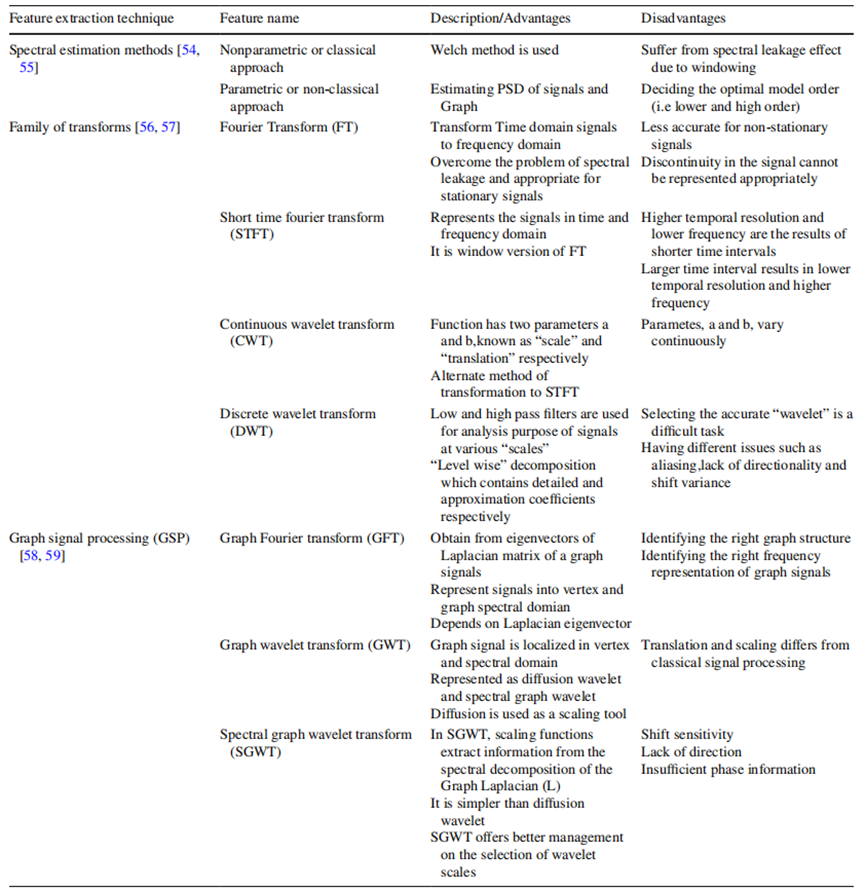
01
time domain analysis
Time domain analysis of EEG signals involves examining changes in signal characteristics over time. This includes understanding the amplitude, duration, and shape of various EEG waveforms.
-
Amplitude analysis: measures the strength of the EEG signal. High amplitude may indicate strong neural activity, while low amplitude may indicate that the brain is in a relaxed or inactive state;
-
Temporal patterns: include recurring patterns in the EEG signal, which may indicate regular brain activity or abnormal patterns such as seizures;
-
Statistical measures: variability, mean, standard deviation, skewness and kurtosis are statistical measures applied to EEG to understand its distribution and characteristics.
02
frequency domain analysis
Aims to reveal potential frequency components in EEG signals. Different brain activities are expressed in different frequency bands.
-
Power Spectral Density (PSD): describes how power is distributed over various frequencies, which is calculated using techniques such as the Fast Fourier Transform (FFT) and provides insight into the primary frequency band;
-
Band Power: Analyzing power within the EEG band can provide specific information about the state of the brain.
03
Time-frequency domain analysis
The EEG signal is dynamic, showing changes in frequency over time, highlighting the importance of time-frequency analysis.
-
Short Time Fourier Transform (STFT): the STFT allows us to observe changes in the frequency content of an EEG signal over a short period of time. By using windows of different sizes, it provides time localized frequency analysis, making it suitable for non-stationary signals;
-
Continuous Wavelet Transform (CWT): analyzes signals on different scales and provides high resolution in the time and frequency domains. It is particularly useful for capturing transient events and frequency changes over different time intervals;
-
Spectrogram: illustrates how the spectrum of a signal changes over time; darker areas on a 3D spectrogram indicate higher power or amplitude in a specific frequency range at a specific time.








reprocess
Feature selection and dimensionality reduction techniques are types of post-processing. To construct a model, a subset of relevant features must be selected using feature selection techniques. Feature selection eliminates irrelevant variables or noise, reduces computational complexity, reduces overfitting, and improves the generalization of the model. Dimensionality reduction (sometimes called feature extraction) combines features to extract a new set of features. Reducing the dimensionality or features of the EEG signal is required to interpret the data and obtain more accurate results as well as to improve classification accuracy. Using feature extraction, high-dimensional data is converted to lower dimensions.








Analysis of results
EEG signal analysis can be performed using various machine learning algorithms such as supervised, unsupervised, deep learning neural networks and GSP (Graph Signal Processing) techniques. These techniques combined with EEG signals can diagnose neurological disorders such as seizures. Monitoring other applications such as mood monitoring, sleep stage classification etc.
04
EEG signal classification methods
05
Research gaps and future directions
06
Image signal processing of EEG signals
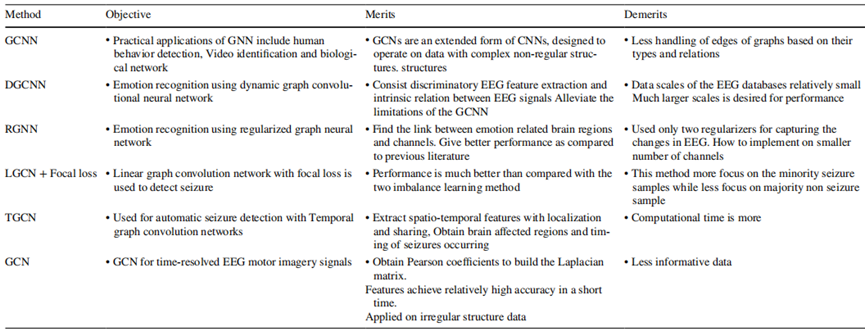
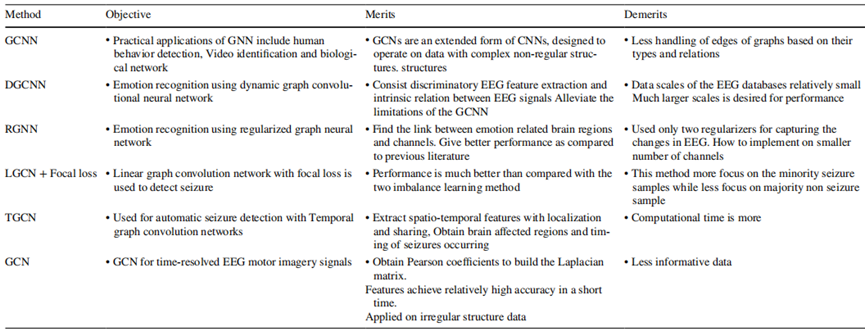
summarize
This study explores different EEG applications, compares data acquisition methods, and scrutinizes signal processing techniques: preprocessing, feature extraction, and post-processing. This paper provides an overview of EEG signal analysis techniques and discusses the challenges in EEG analysis.The rise of GSPs marks a breakthrough in understanding graph-structured biological data. Despite the challenges, these graph-based methods have great potential in scientific fields such as bioengineering.
Exploring the potential impact of EEG signals on future research and clinical practice holds significant promise. In the research arena, further studies could delve into improving signal processing techniques, increasing spatial resolution, and exploring advanced machine learning algorithms for more precise and nuanced interpretations. On the clinical side, the use of EEG signals may guide the development of personalized treatment plans, improved diagnostic tools and real-time monitoring of neurological disorders.
Original Message
Sharma Ramnivas,Meena Hemant Kumar. Emerging Trends in EEG Signal Processing: a Systematic Review[J]. SN Computer Science,2024,5(4).
Introduction to Portable EEG Systems
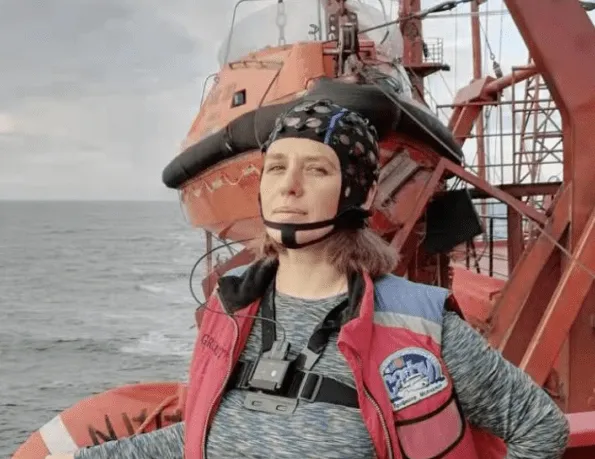
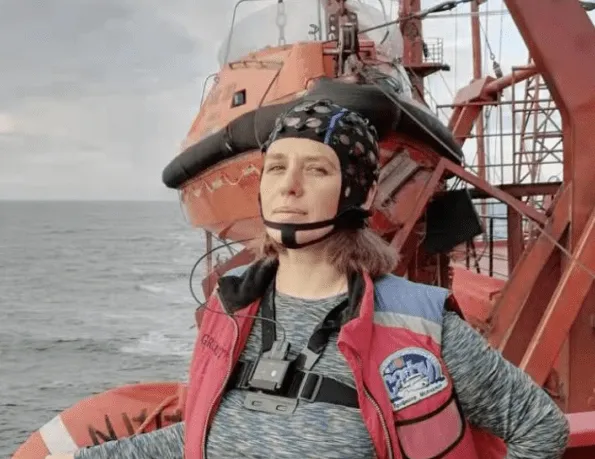
Mitsar is a company that produces high-quality EEG amplifiers for capturing, displaying and storing the electrical activity of the user's brain.
The SmartBCI Portable EEG System is a wireless wearable portable EEG with dry electrodes, conductive paste, and other acquisition methods for real-world data collection.The SmartBCI Portable EEG System includes a multi-channel, professional-grade, wireless wearable EEG amplifier, accessories, and an advanced software package.Can be applied toGodEngineering Management, Psychology, RecognitionKnowing Neuroscience,Research in human factors engineering, human-computer interaction, human-computer-environment, cognitive neuroscience, brain science, and brain-computer interfaces.
The electrode caps are arranged in the standard international 10-20 arrangement, and users can choose from a variety of collection methods such as conductive paste and saline depending on their needs. Medium electrode caps are generally suitable for users over 5 years old. Small electrode caps are generally suitable for children between the ages of 2 and 5. Extra Small Electrode Caps are typically used for children 9 months to 2 years of age. (Age sizes are recommendations and should be based on actual head circumference).
Introduction to the 41-channel EEG system
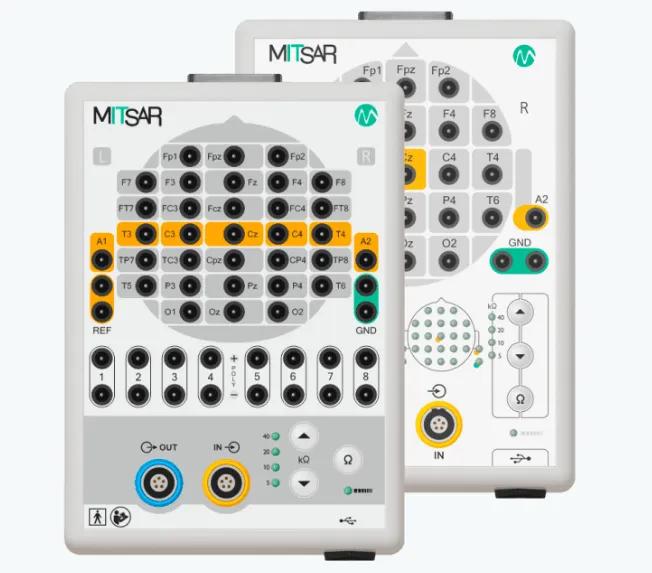
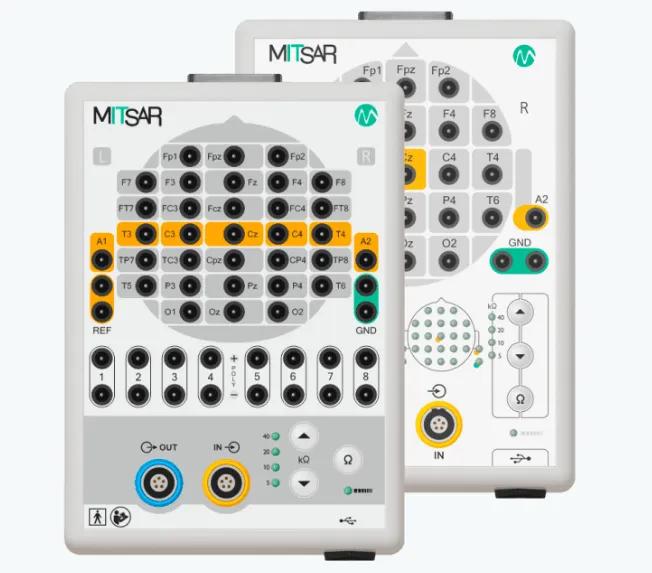
Mitsar The EEG-202 device is a 41-channel physiological acquisition device with 33 EEG channels and 8 bipolar channels. In addition, theThe acquisition system can also be used for video EEG monitoring and evoked (event-related) potentials, and the software has EEG/ERP/QEEG acquisition and analysis functions.Can be applied toGodEngineering Management, Psychology, RecognitionKnowing Neuroscience,Research in human factors engineering, human-computer interaction, human-computer-environment, cognitive neuroscience, brain science, and brain-computer interfaces.
Company Profile
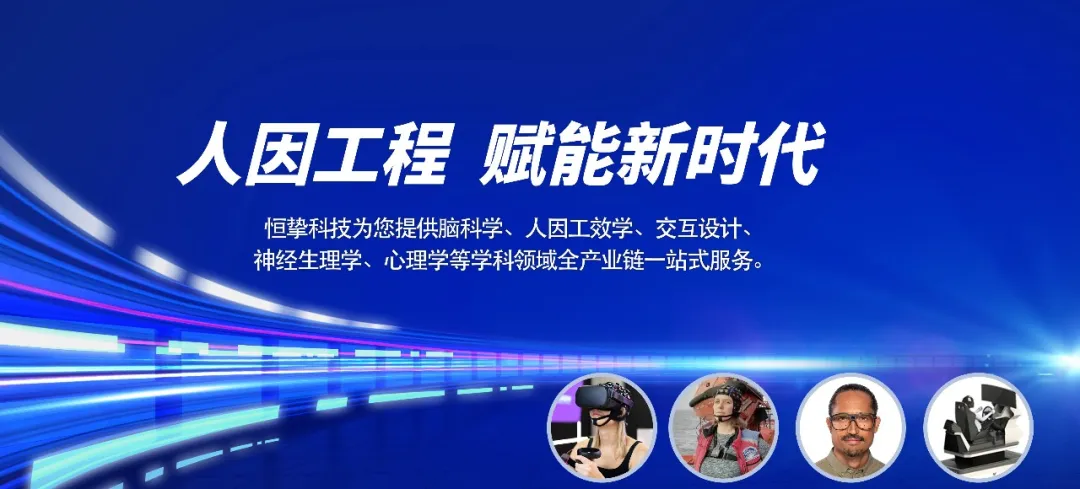
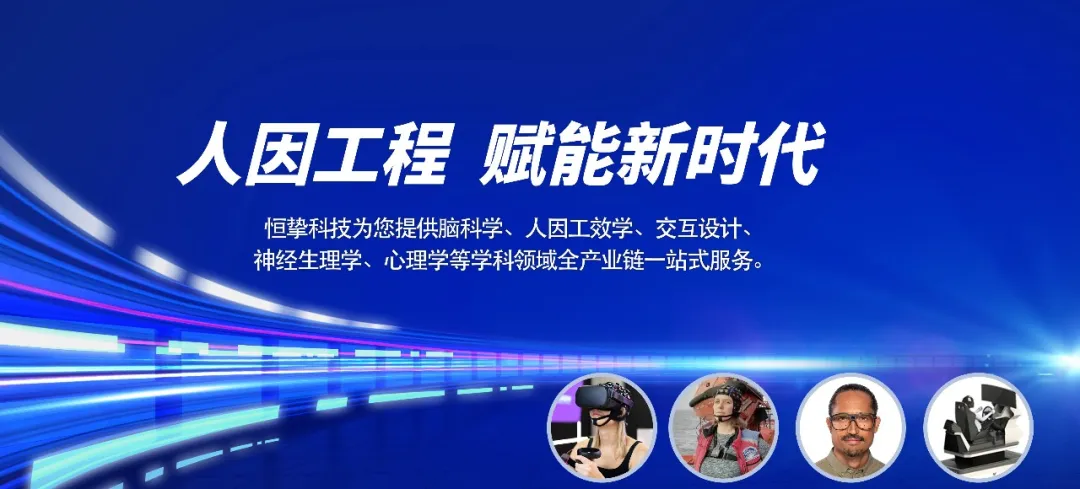
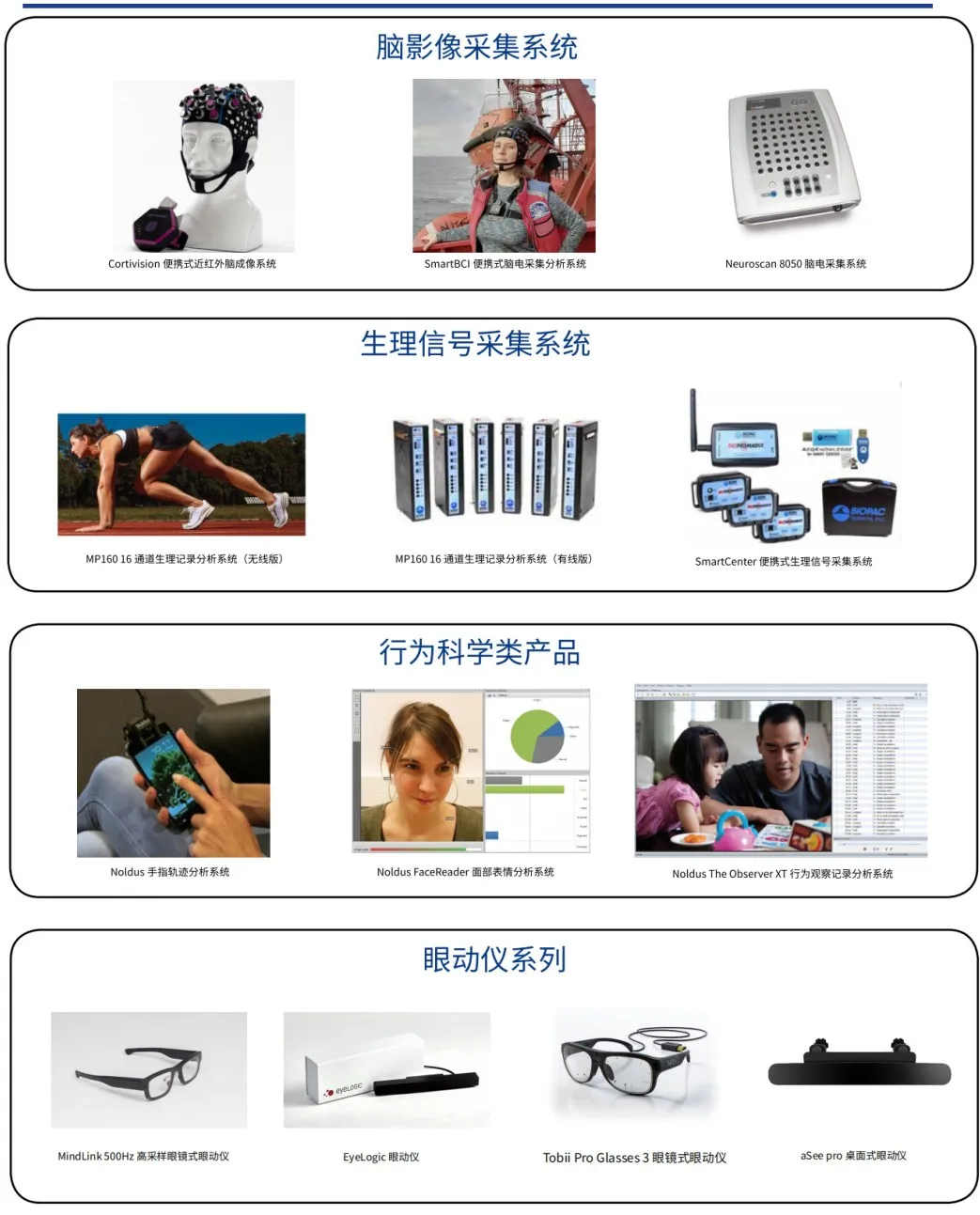
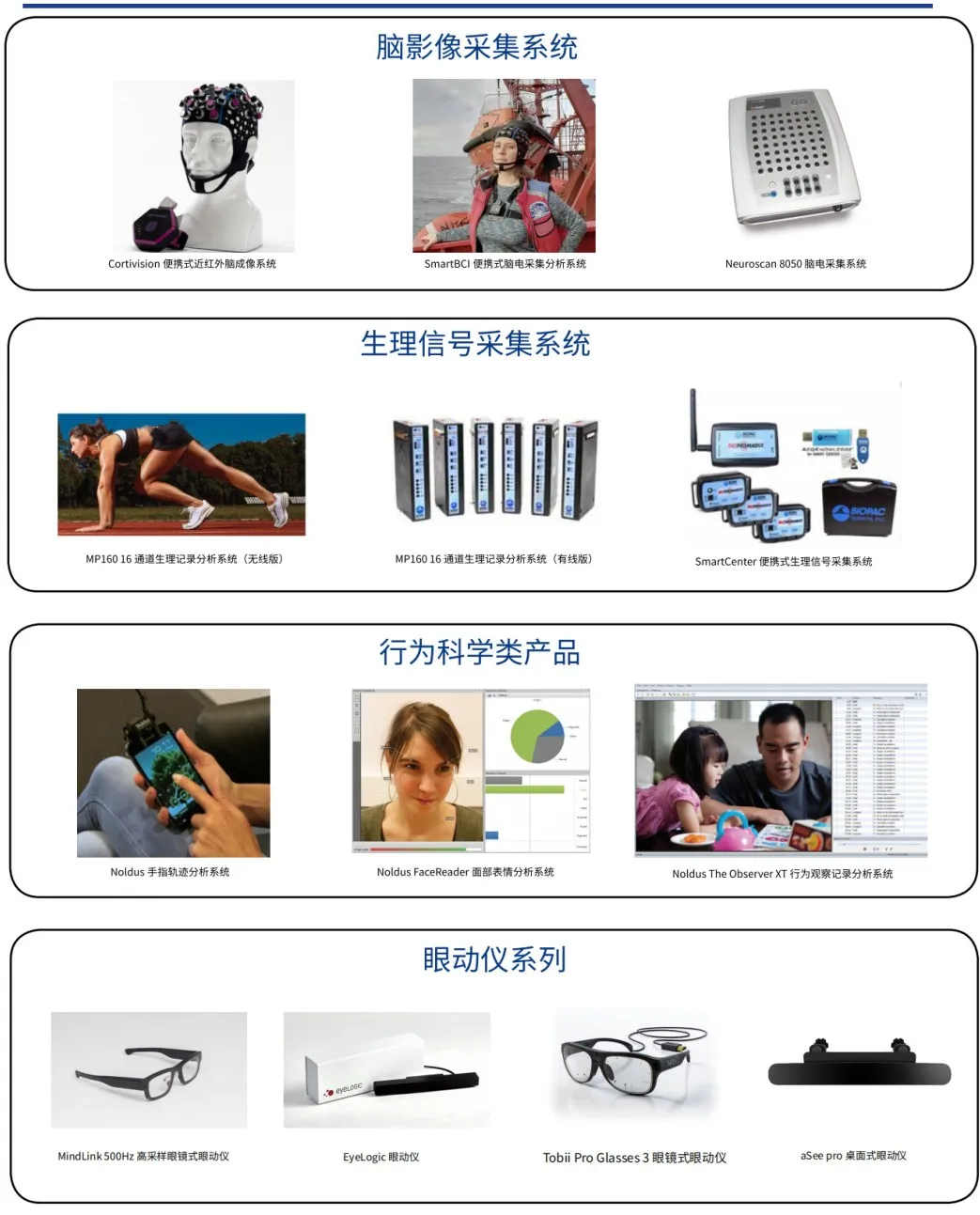
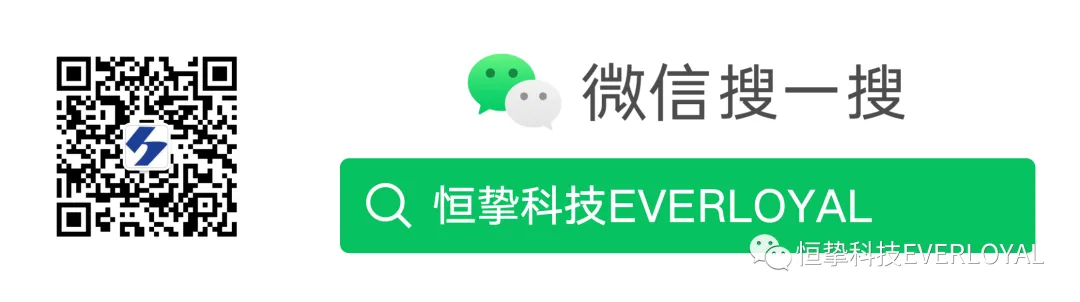
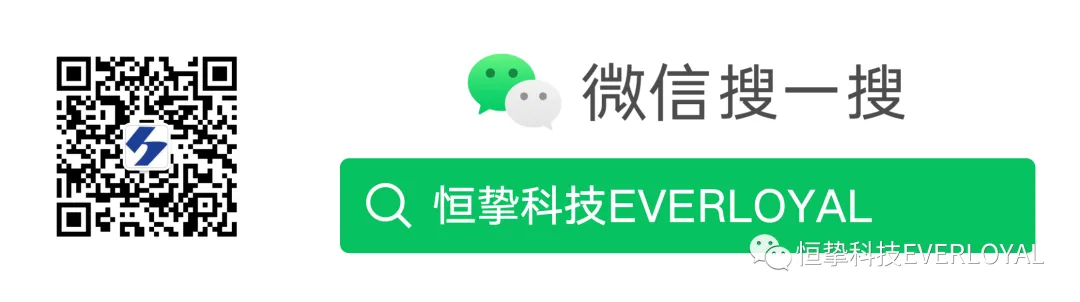