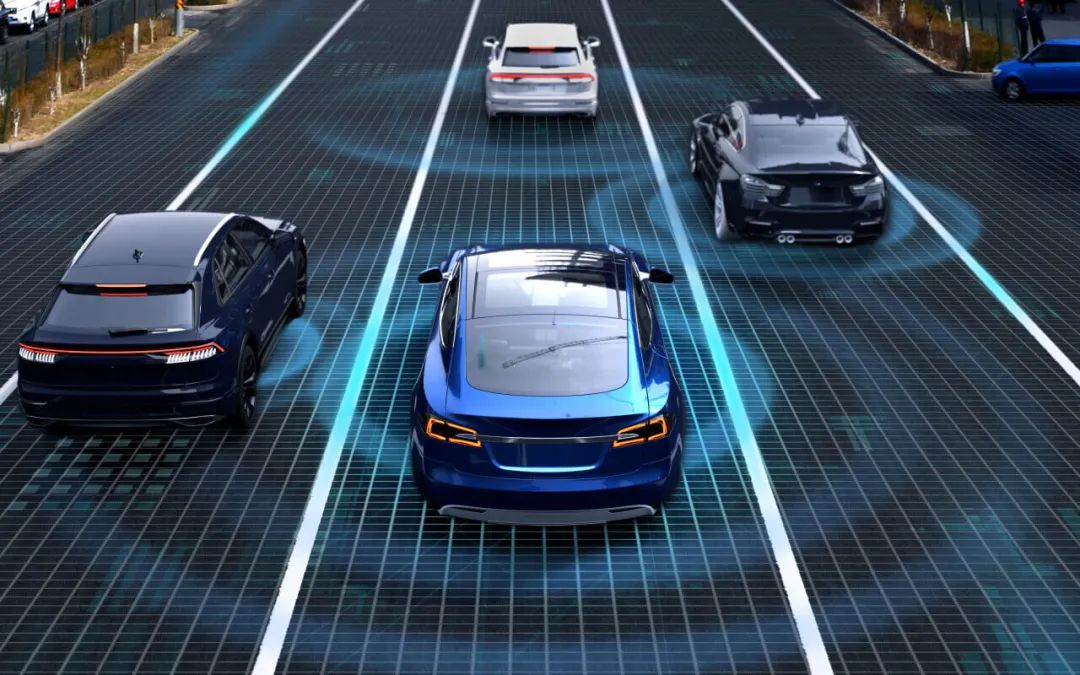
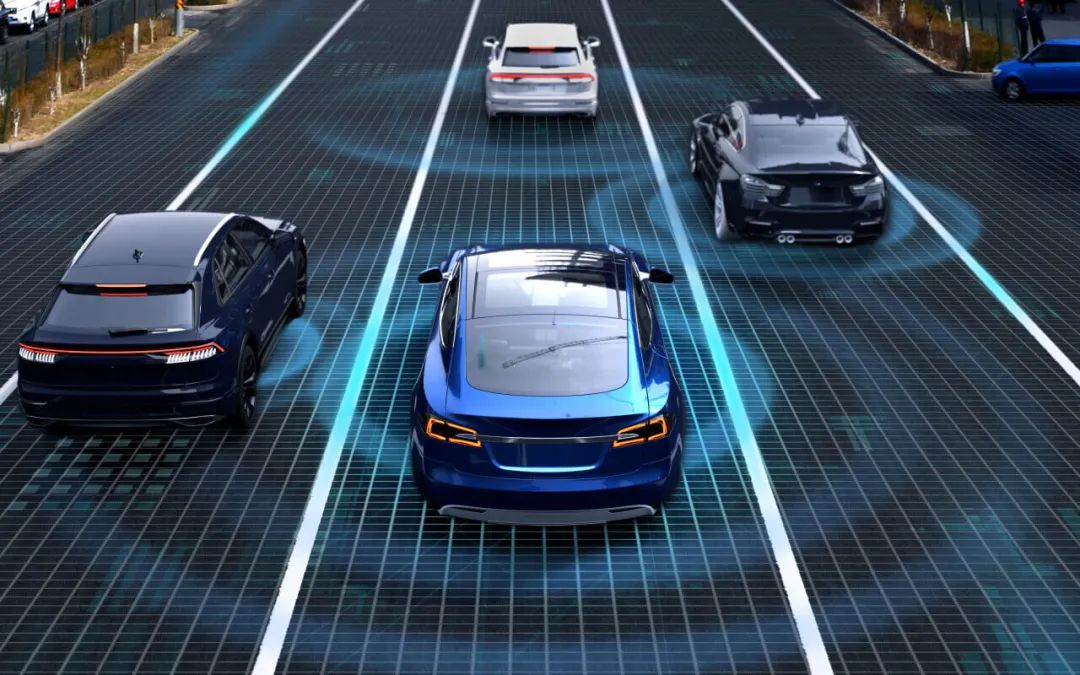
introductory
Autonomous vehicles (AVs) have the potential to reduce road traffic accidents, improve traffic efficiency and reduce fuel consumption. In addition, they promise to provide greater mobility for those who are unable to drive due to age or disability. However, a key issue faced is establishing and calibrating driver trust in this technology, as trust plays an important role in determining whether or how a driver makes an AV.
Low trust is reported to be a major factor in discouraging drivers from embracing AVs. The study found that mistrust was the main factor contributing to the low willingness to use AVs. In addition, excessive trust may lead to misuse and abuse of the technology, and may also compromise driver readiness to regain manual control, leading to longer reaction times and increased accident risk. To ensure the safe introduction of AVs into road traffic, it is crucial to develop AVs that can dynamically adapt to the driver's task level in real time, which requires the use of reliable human trust assessment models. The aim of this study is to develop an EEG-based trust assessment model through the use of machine learning algorithms that utilize EEG signals captured by the driver while interacting with a simulated autopilot system that may be malfunctioning.
01
Related work
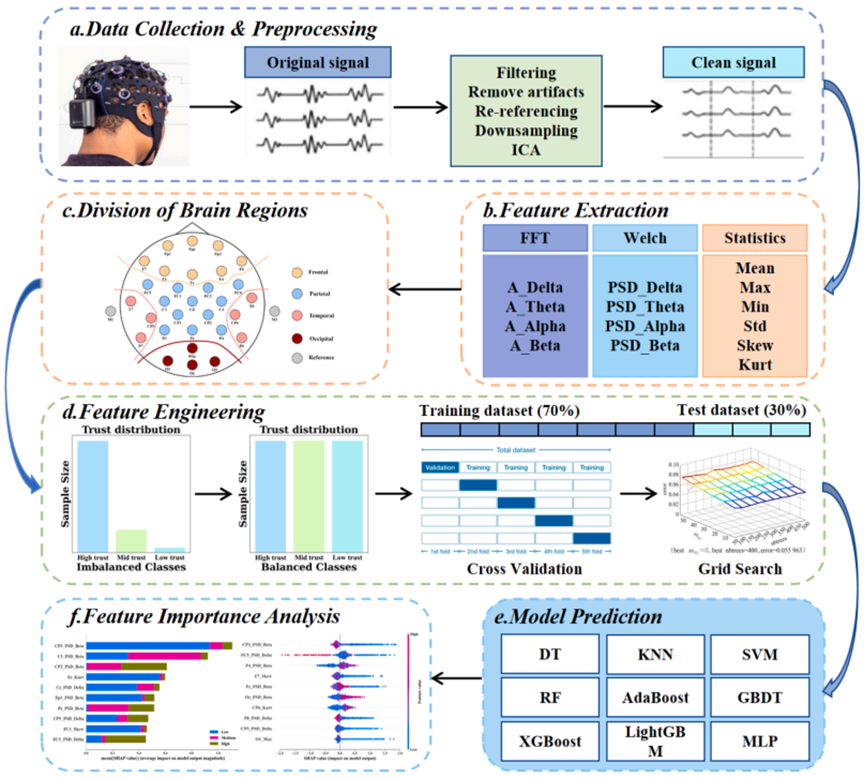
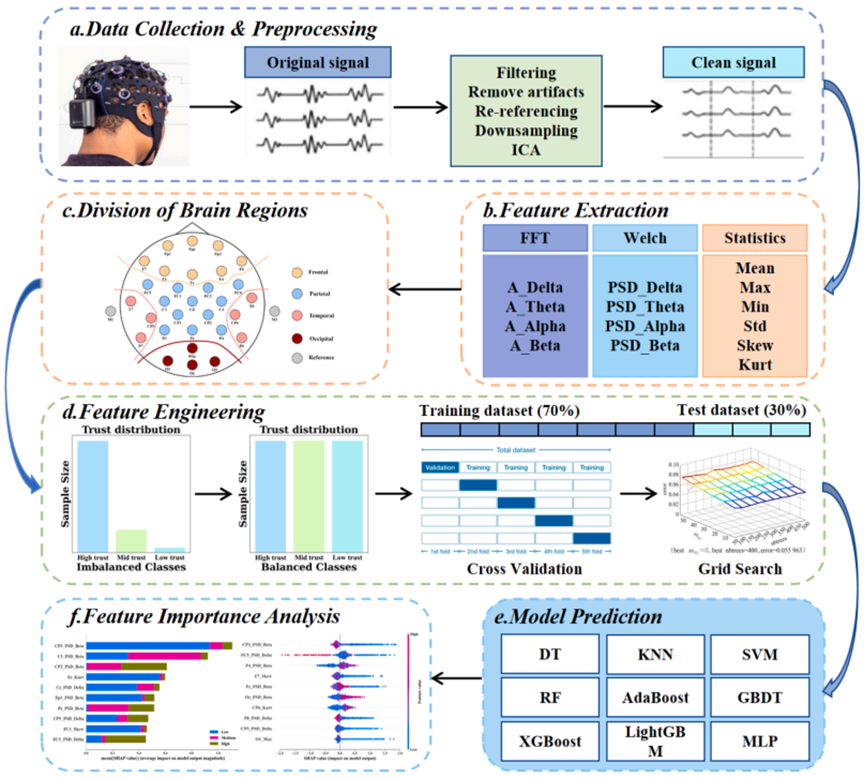
02
Driving Experiment
01
subject's choice
A total of 71 college students (36 males), with a mean age of 22.6 years (SD = 1.6), participated in the driving experiment . All subjects had a valid driver's license and normal or corrected-to-normal vision. Subjects were randomly assigned to one of three experimental conditions: normal, miss, and false alarms, with 24 (12 men and 12 women) in each condition.
02
Experimental equipment
The figure below outlines the driving simulator and EEG recording equipment used in the experiment. The driving simulator consists of three 27-inch LED display flats, a high-performance computer, and other components. Driving data such as speed, acceleration, and lane position were recorded at 60 Hz. The simulator can be run in manual or automatic mode. The EEG consists of 32 channels and records signals at a frequency of 500 Hz, and the contact impedance between the electrodes and the subject is kept below 20 kΩ.
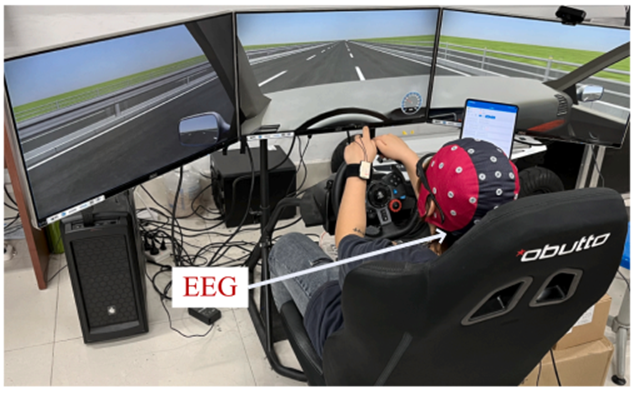
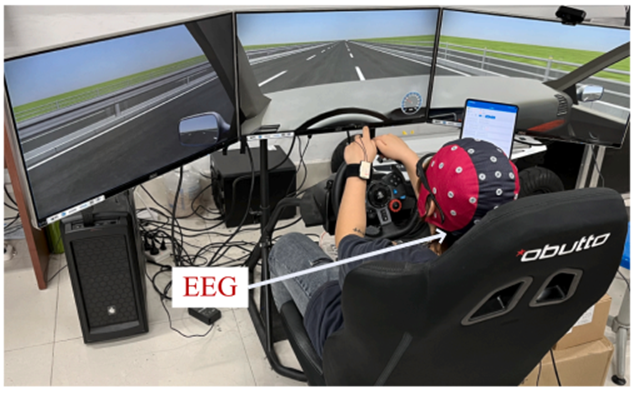
03
Experimental design
04
Driving scenarios and NDRT
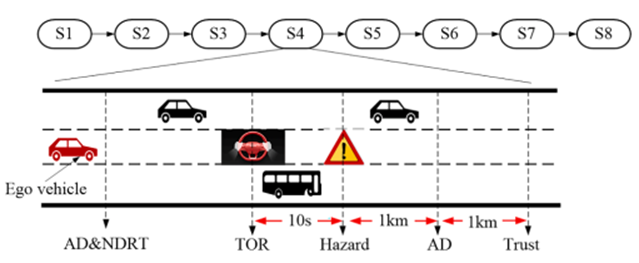
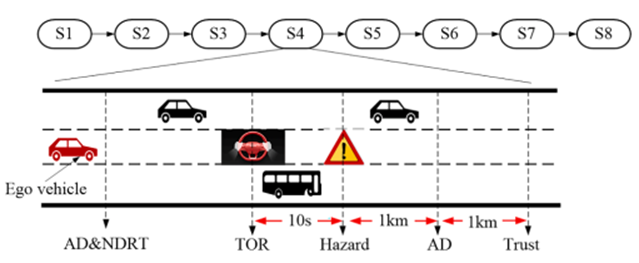
05
experimental procedure
03
data processing





preprocessing
1. Use FIR bandpass filtering (1-30Hz) to remove noise;
2. The signal will be re-referenced to an average reference;
3. Use the FASTER toolbox to identify and mark bad guides, then use spherical interpolation to remove them;
4. Independent principal component analysis (ICA) was performed to decompose the EEG signal, and the ADJUST toolbox was applied to identify and remove artifacts.





Trust labeling and feature extraction
The EEG signals recorded at a distance of 1Km before the trust rating, corresponding to a duration of about 45s, are used for feature extraction, and model development. Each scene is extracted for about 5 cycles.
Verbal scores in the range of [0,60] are labeled as "low trust", [60,80] as "medium trust", and [80,100] as "high trust". ".
Six time-domain features were calculated for the EEG signal, including mean, maximum, minimum, standard deviation, skewness and kurtosis. To calculate the frequency domain features, the EEG signal was divided into four bands: delta (1-4Hz), theta (4-8Hz), alpha (8-13Hz) and beta (13-30hz). The amplitude and power spectral density (PSD) of each band were then calculated using FFT and Welch algorithms, respectively.





Sorter Overview
The samples were allocated as training and test sets in a ratio of 70-30. To construct the trust assessment model, nine classifiers were used: dt, KNN, SVM, RF, adaptive boosting (AdaBoost), gradient boosting decision tree (GBDT), XGBoost, LightGBM, multilayer perceptron (MLP).





Indicators of achievement
In order to fully evaluate the performance of the model under unbalanced multiclassification problems, four metrics derived from the confusion matrix species, i.e., accuracy, precision, recall, and F1 score, are calculated. The four metrics expressions are given below:
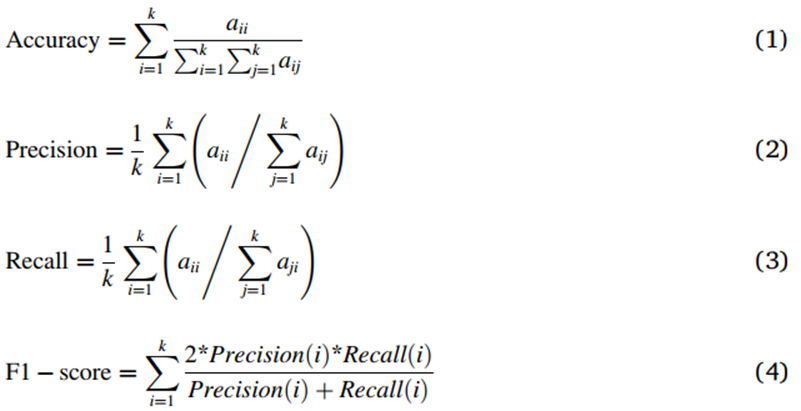
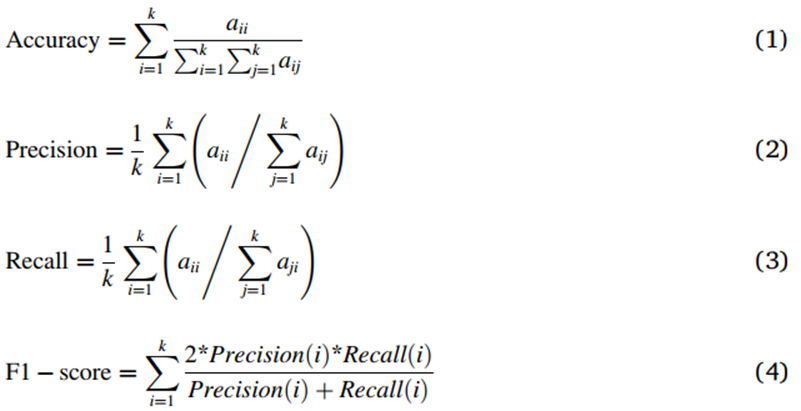
04
Results
01
Whole-brain based trust assessment model
Using the FEF method, a total of 300 features were selected from the whole-brain regional feature set species to build a trust assessment model.
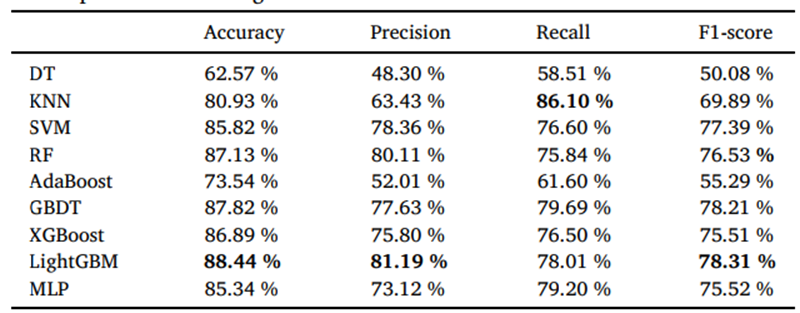
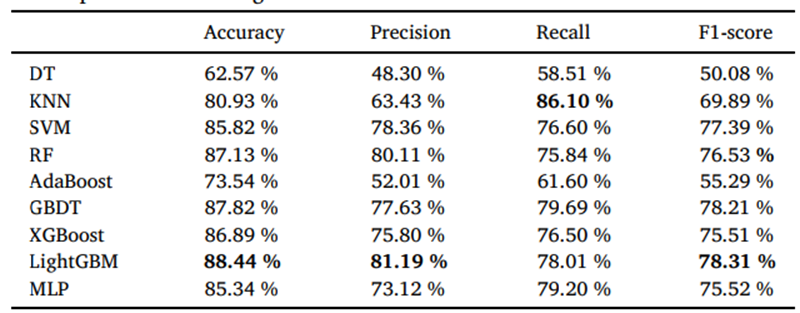
02
A model for trust assessment based on individual brain regions
The 32 electrodes were divided into 4 brain regions as shown in Figure 4 below.The REF method selected 74, 72, 40 and 86 features from frontal, temporal, occipital and parietal regions respectively.
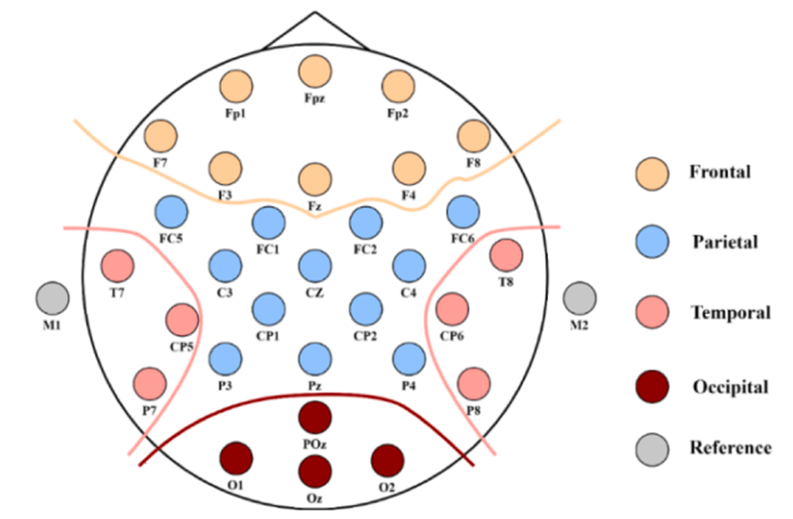
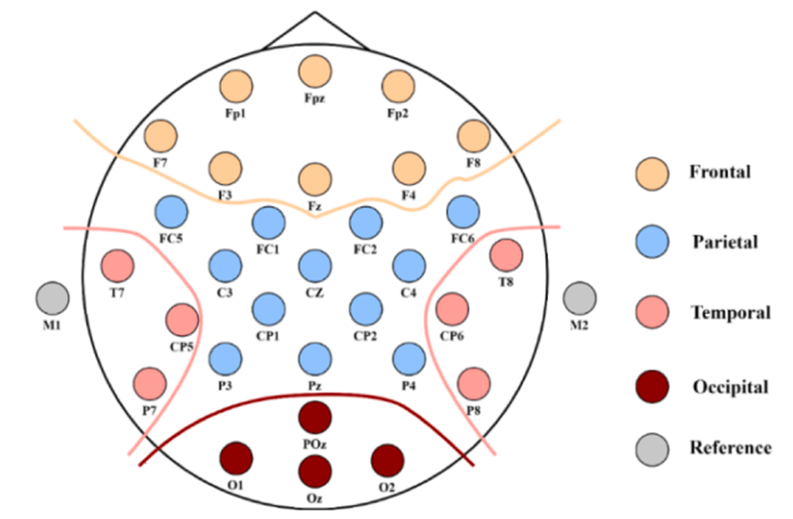
These features were used to develop a trust model with 9 classifiers.
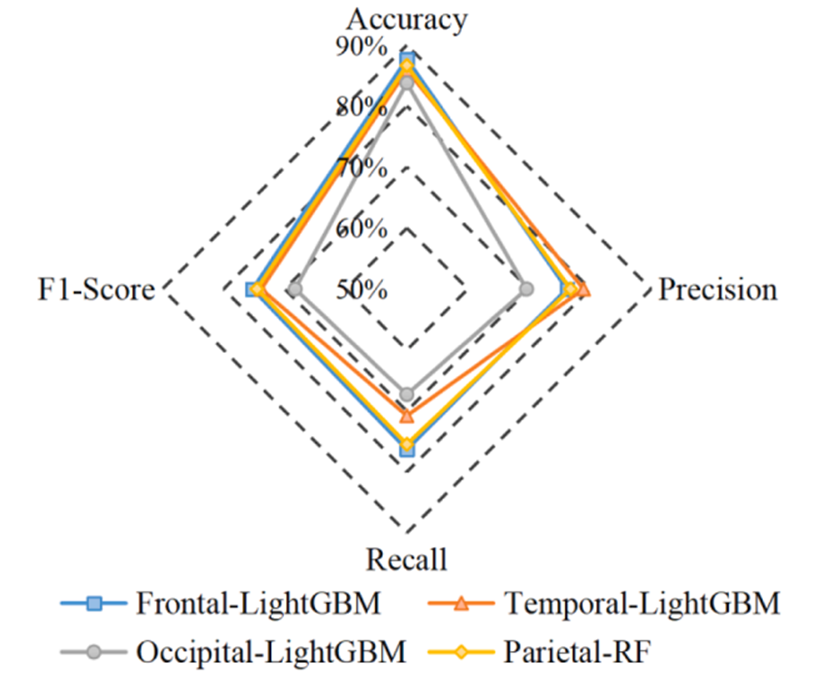
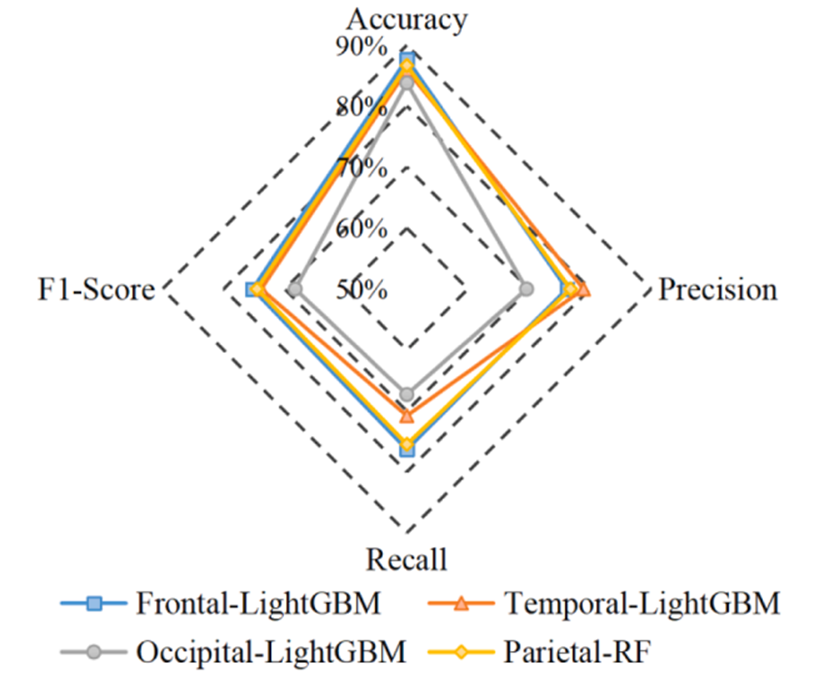
03
Comparing brain region performance in trust models
The figure below shows the average performance metrics for the 9 classifiers for the entire brain region. The whole brain overall showed better performance compared to individual brain regions. Frontal and parietal regions performed significantly better than occipital regions for all 4 performance metrics. Frontal regions outperformed temporal regions in accuracy (p = 0.044).
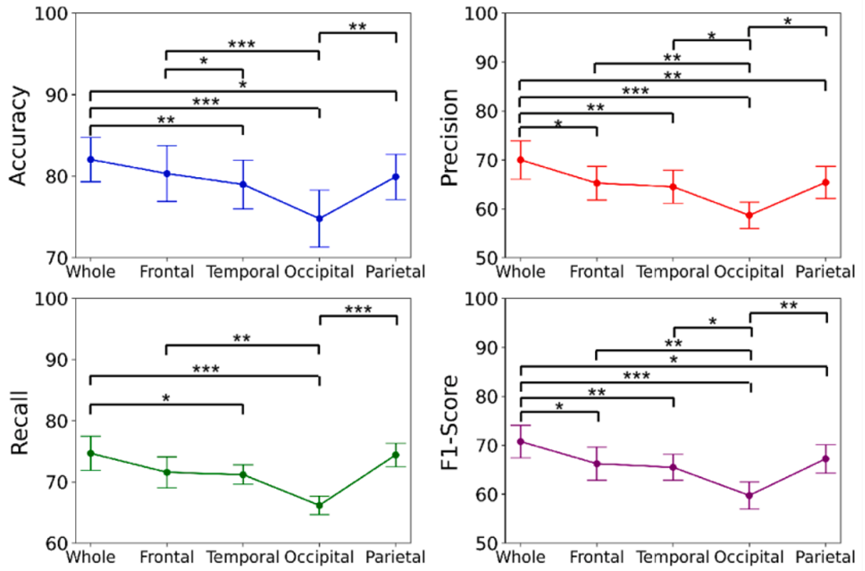
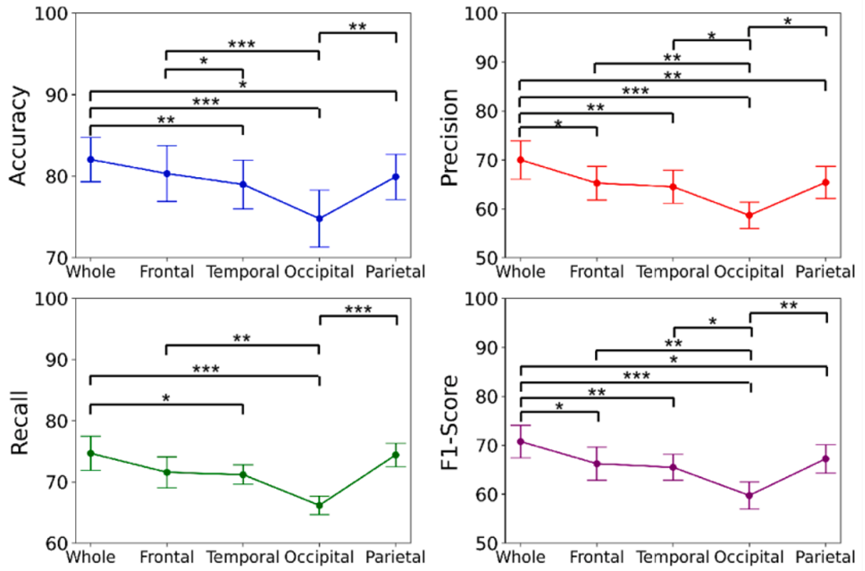
04
Confusion Matrix and Characteristic Importance Analysis
Based on the above results, the LightGBM model using whole-brain features was used. First, the confusion matrix of the model was pushed to. The results showed that the model effectively learned the categorization boundaries between low and medium trust and between ground trust and high confidence.
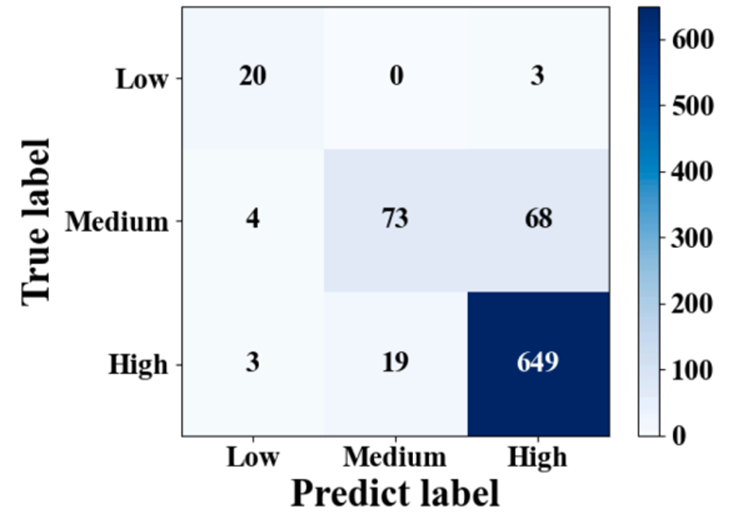
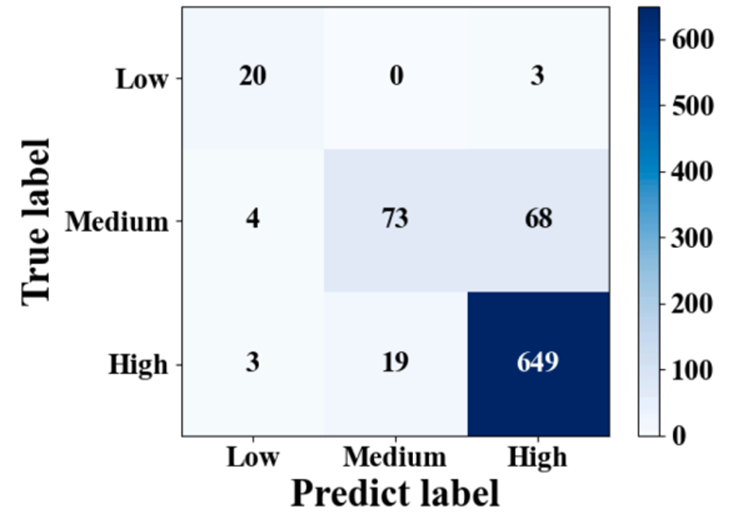
The SHAP method was used to calculate the feature importance values. The top 10 features that contribute the most to the model are given in the following figures respectively. Five of the eight PSD features are from the beta wave, which suggests that PSD of the beta wave has a key role in trust assessment. Most of the 10 belong to the parietal lobe from the brain region perspective.
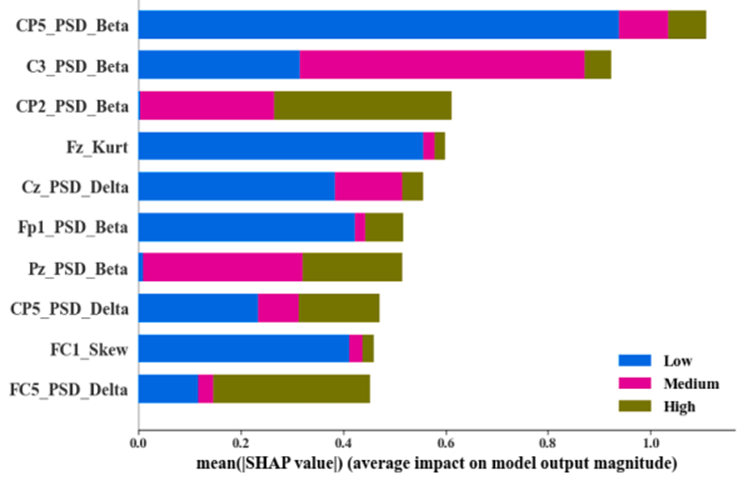
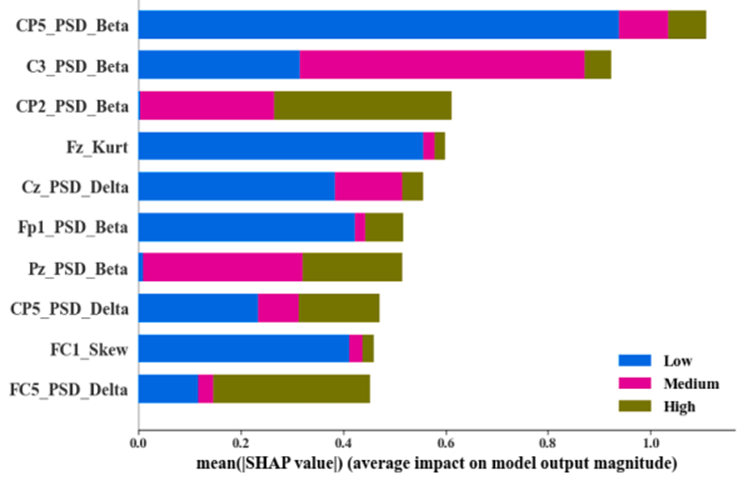
summarize
Assessing driver trust is a major challenge in the development of adaptive self-driving cars. EEG directly measures brain electrical activity, which more directly reflects the neural processes related to trust. In addition, the high temporal resolution of EEG can capture rapid changes in neural activity in a very short period of time, which is crucial for detecting dynamic trust changes in human-AV interactions in real time.
This study is a preliminary attempt to model trust assessment based on large-scale EEG signals. The best performing model utilized whole-brain features with an accuracy of 88.441 TP3T and an F1 score of 78.311 TP3T using the LightGBM algorithm.These results support the feasibility of using EEG features to predict driver trust. In addition, models constructed using only features from frontal or parietal regions showed competitive performance, not only with the potential to develop cost-effective models, but also with a reduced number of features. The study also characterized the best performing models and found that increased beta power tended to indicate lower levels of trust in the AV.
Introduction to Portable EEG Systems
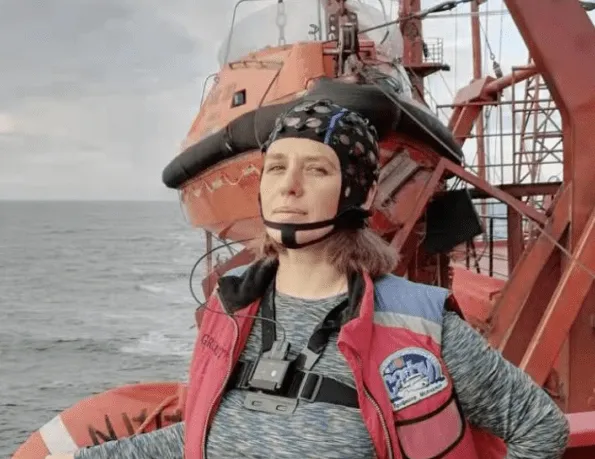
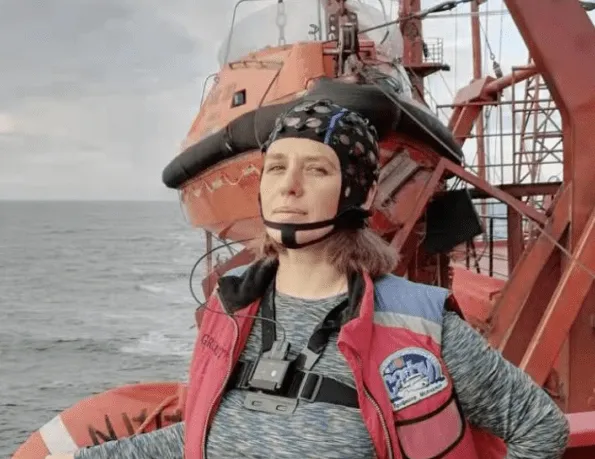
Mitsar is a company that produces high-quality EEG amplifiers for capturing, displaying and storing the electrical activity of the user's brain.
The SmartBCI Portable EEG System is a wireless wearable portable EEG with dry electrodes, conductive paste, and other acquisition methods for real-world data collection.The SmartBCI Portable EEG System includes a multi-channel, professional-grade, wireless wearable EEG amplifier, accessories, and an advanced software package.Can be applied toGodEngineering Management, Psychology, RecognitionKnowing Neuroscience,Research in human factors engineering, human-computer interaction, human-computer-environment, cognitive neuroscience, brain science, and brain-computer interfaces.
The electrode caps are arranged in the standard international 10-20 arrangement, and users can choose from a variety of collection methods such as conductive paste and saline depending on their needs. Medium electrode caps are generally suitable for users over 5 years old. Small electrode caps are generally suitable for children between the ages of 2 and 5. Extra Small Electrode Caps are typically used for children 9 months to 2 years of age. (Age sizes are recommendations and should be based on actual head circumference).
Introduction to the 41-channel EEG system
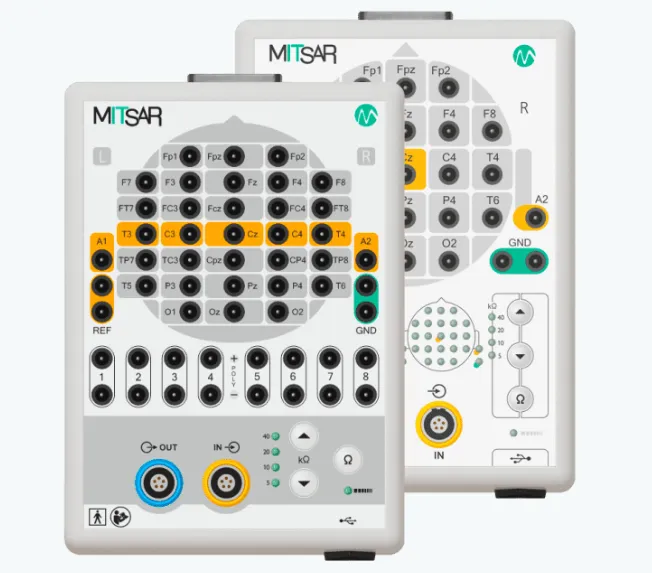
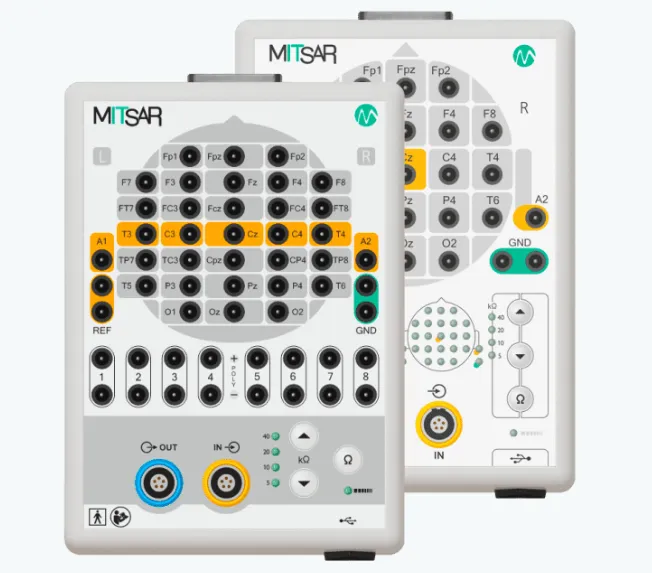
Mitsar The EEG-202 device is a 41-channel physiological acquisition device with 33 EEG channels and 8 bipolar channels. In addition, theThe acquisition system can also be used for video EEG monitoring and evoked (event-related) potentials, and the software has EEG/ERP/QEEG acquisition and analysis functions.Can be applied toGodEngineering Management, Psychology, RecognitionKnowing Neuroscience,Research in human factors engineering, human-computer interaction, human-computer-environment, cognitive neuroscience, brain science, and brain-computer interfaces.
Company Profile
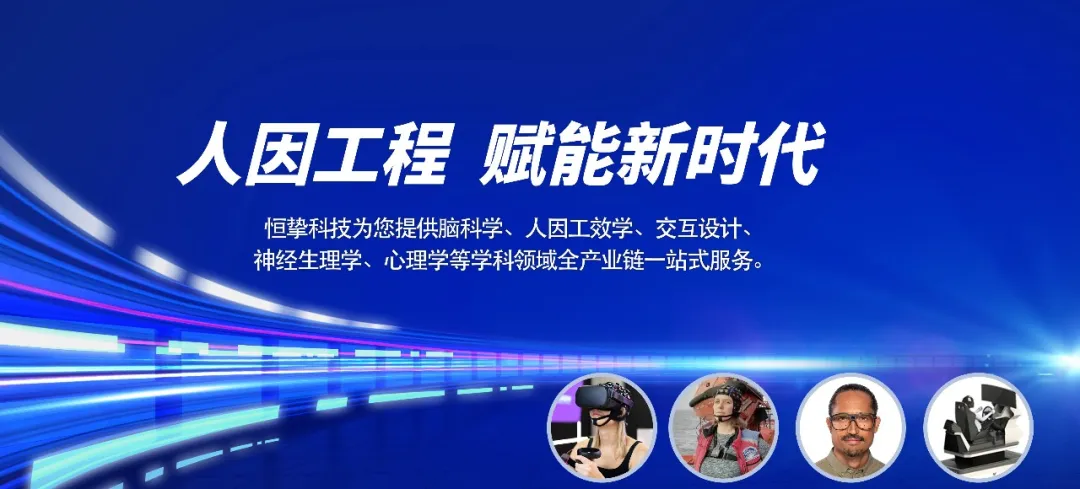
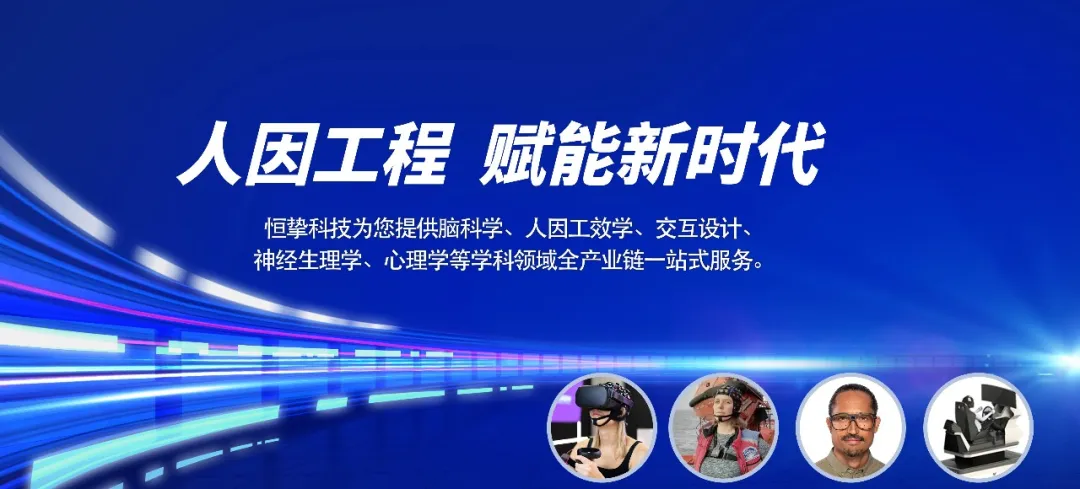
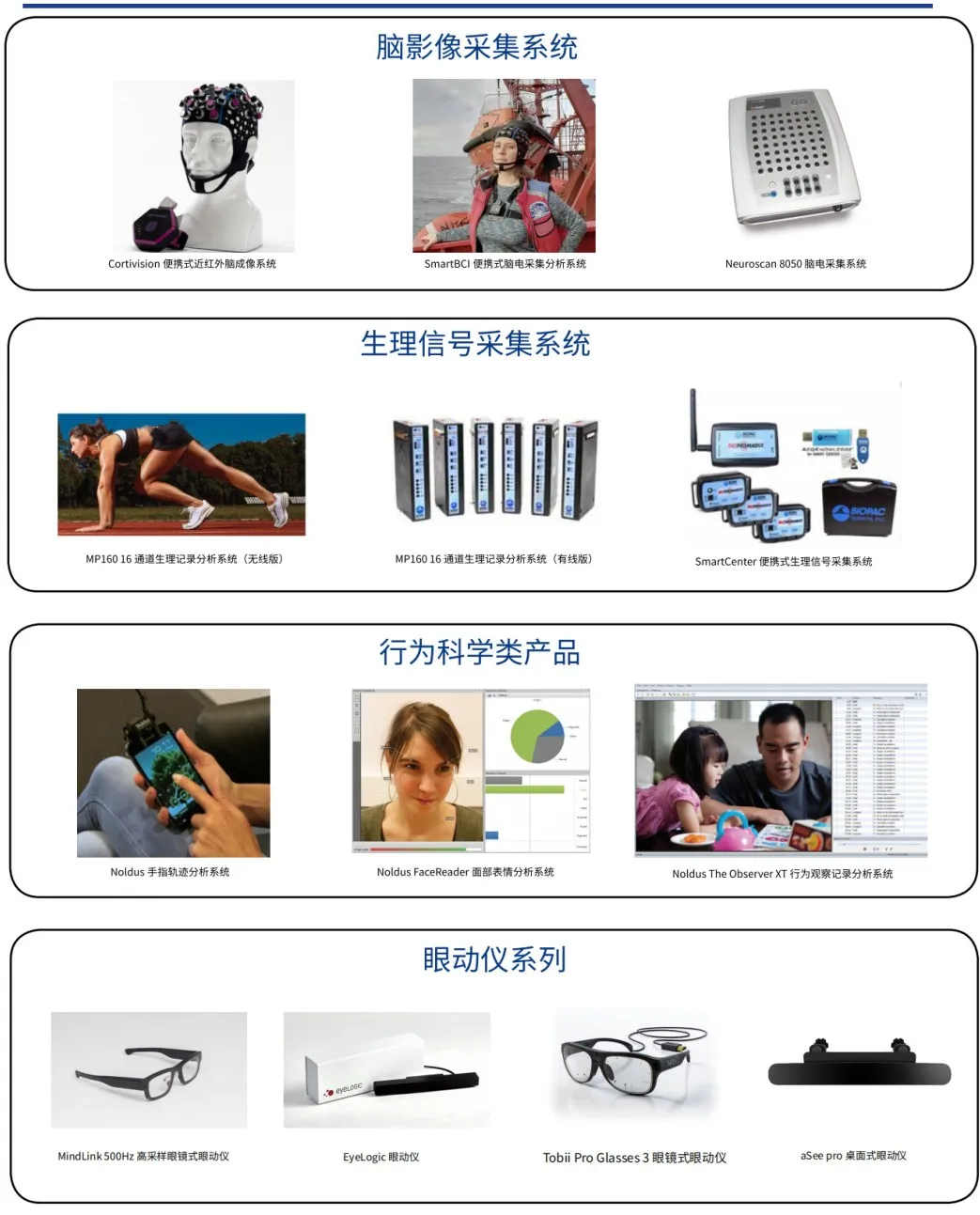
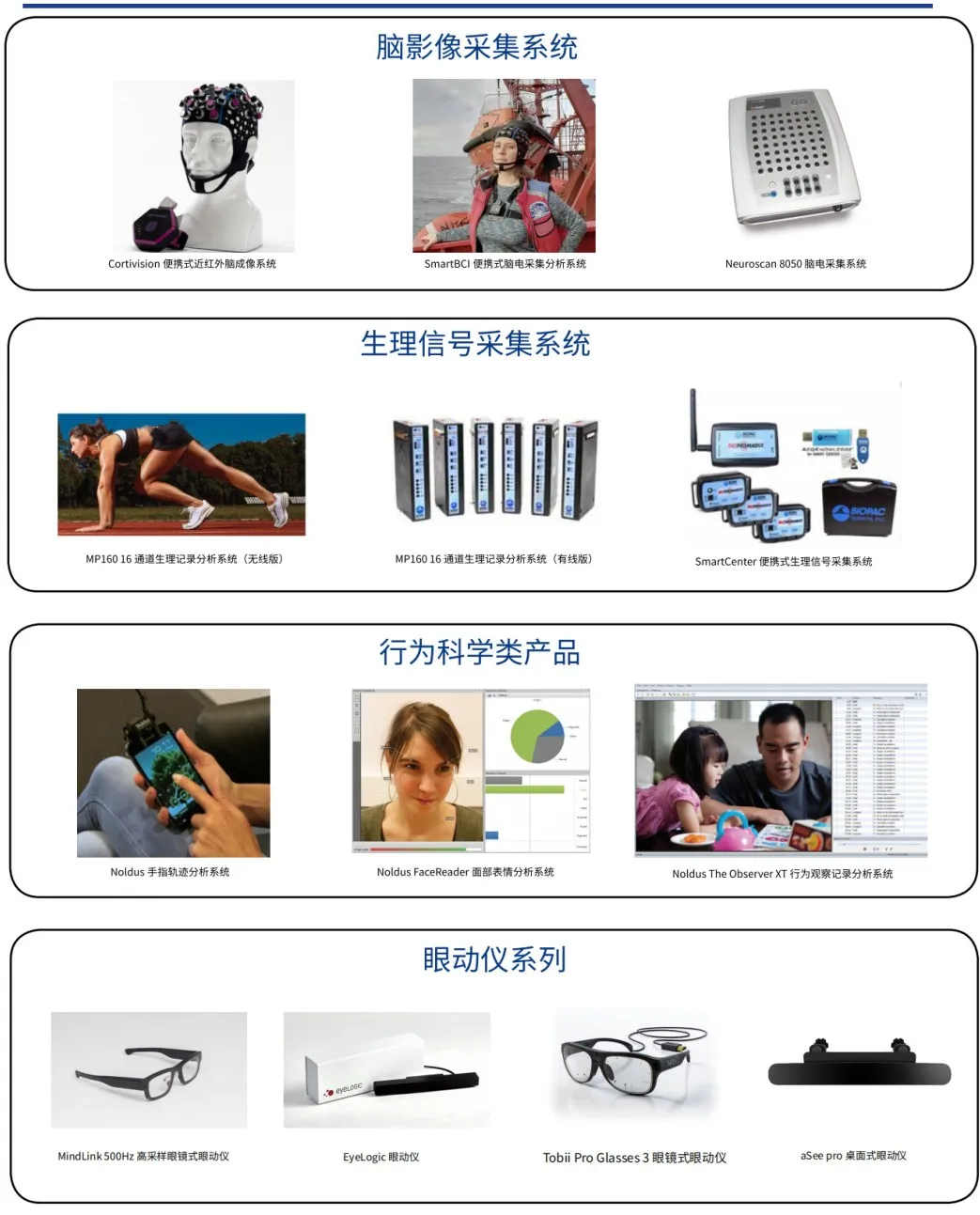
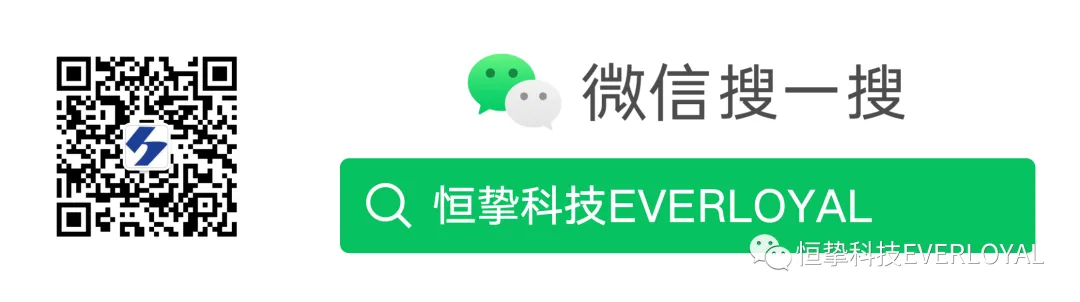
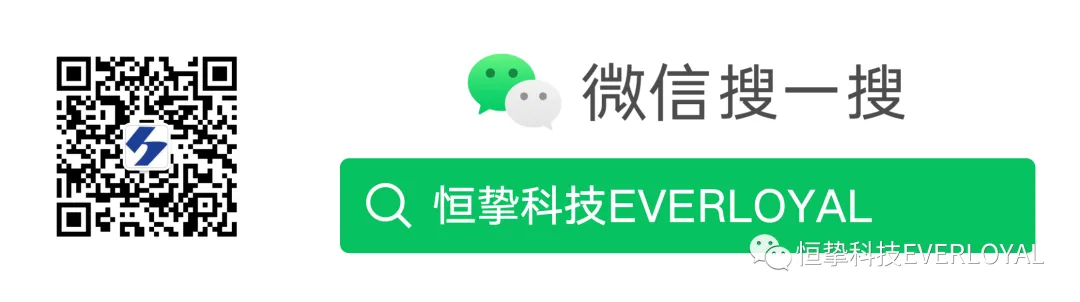