The structure and function of the brain is the most challenging scientific issue in the 21st century, and the related scientific knowledge can not only analyze the generation and operation of the human mind, but also effectively integrate with information and communication technology, giving rise to artificial intelligence, brain-like intelligence, digital society and other emerging industries. China has put forward the layout of China's brain program "with the neurological basis of brain cognition as the main body" and "with brain diseases and brain intelligence as the two wings", in which the brain-computer interface is the key technology of "brain-like intelligent computing and brain-computer intelligence". Brain-computer interface is the key technology of "brain-like intelligent computing and brain-machine intelligence". In this regard, the Bureau of Academic Affairs of the Chinese Academy of Sciences (CAS) has set up a consulting project entitled "Brain-computer Interface Key Scientific Issues, Key Core Technologies and Their Layout Research" led by Professor Cheng Heping, academician of CAS and professor of Peking University, in order to comprehensively sort out and analyze the key scientific issues and technological challenges of the Brain-computer Interface as well as the related development paths.
Under the promotion of Academician Cheng Heping and Dr. Wang Zhenyu, the guest editor of this journal, Science and Society has organized and published the topic of "Brain-Computer Interfaces", which discusses the relationship between brain-computer interface technology and society from different perspectives, such as the progress and prospect of brain-computer interface technology, the social impact, the ethical risk, and the public governance, and so on. It is hoped that the publication of this topic can attract people from all walks of life to pay attention to brain-computer interfaces, and work together for the healthy development of brain-computer interface technology, as well as to better serve the society and improve human welfare.


Current status of brain-computer interface technology development
and future prospects
[1] |
Zander TO, Kothe C. Towards Passive Brain-computer Interfaces: Applying Brain-computer Interface Technology to Human-machine Systems in General. Journal of Neural Engineering, 2011, 8(2): 025005. DOI: 10.1088/1741-2560/8/2/025005. |
[2] |
Wolpaw JR, Birbaumer N, Heetderks WJ, et al. Brain-computer Interface Technology: A Review of the First International Meeting. IEEE Transactions on Rehabilitation Engineering: A Publication of the IEEE Engineering in Medicine and Biology Society, 2000, 8(2): 164-173. DOI: 10.1109/TRE.2000. 847807. |
[3] |
Mudgal SK, Sharma SK, Chaturvedi J, et al. Brain Computer Interface Advancement in Neurosciences: Applications and Issues. Interdisciplinary Neurosurgery, 2020, (20): 100694. |
[4] |
|
[5] |
|
[6] |
Wang Y, Wang R, Gao X, et al. A Practical VEP-based Brain-computer Interface. IEEE Transactions on Neural Systems and Rehabilitation Engineering: A Publication of the IEEE Engineering in Medicine and Biology Society, 2006, 14(2): 234-239. |
[7] |
Wolpaw JR, McFarland DJ, Neat GW, et al. An EEG-based Brain-computer Interface for Cursor Control. Electroencephalography and Clinical Neurophysiology, 1991, 78(3): 252-259. DOI: 10.1016/0013-4694(91)90040-B. |
[8] |
Abiri R, Borhani S, Sellers EW, et al. A Comprehensive Review of EEG-based Brain-computer Interface Paradigms. Journal of Neural Engineering, 2019, 16( 1): 011001. doi: 10.1088/1741-2552/aaf12e. |
[9] |
Rashid M, Sulaiman N, P P Abdul Majeed A, et al. Current Status, Challenges, and Possible Solutions of EEG-Based Brain-Computer Interface: a Comprehensive Review. Frontiers in Neurorobotics, 2020(14): Article 25. |
[10] |
Silversmith DB, Abiri R, Hardy NF, et al. Plug-and-play Control of a Brain-computer Interface Through Neural Map Stabilization. Nature Biotechnology, 2021, 39(3): 326-335. doi: 10.1038/s41587-020-0662-5. |
[11] |
Saha S, Mamun KA, Ahmed K, et al. Progress in Brain Computer Interface: Challenges and Opportunities. Frontiers in Systems Neuroscience, 2021, (15). 578875. |
[12] |
Chen SG, Chen CJ, Liu BC, et al. Advances in brain-computer interface research in 2022. Signal Processing, 2023, 39(8): 1355-1366. |
[13] |
Farwell LA, Donchin E. Talking Off the Top of Your Head: toward a Mental Prosthesis Utilizing Event-related Brain Potentials. electroencephalography and Clinical Neurophysiology, 1988, 70(6): 510-523. DOI: 10.1016/0013-4694(88)90149-6. |
[14] |
Vidal JJ. Toward Direct Brain-computer Communication. Annual Review of Biophysics and Bioengineering, 1973, 2(1): 157-180. DOI: 10.1146/annurev. bb.02.060173.001105. |
[15] |
Birbaumer N, Ghanayim N, Hinterberger T, et al. A Spelling Device for the Paralysed. Nature, 1999, 398(6725): 297-298. DOI: 10.1038/18581. |
[16] |
Alcaide-Aguirre RE, Warschausky SA, Brown D, et al. Asynchronous Brain-computer Interface for Cognitive Assessment in People with Cerebral Palsy. Journal of Neural Engineering, 2017, 14(6): 066001. doi: 10.1088/1741-2552/aa7fc4. |
[17] |
Shahriari Y, Vaughan TM, McCane LM, et al. An Exploration of BCI Performance Variations in People with Amyotrophic Lateral Sclerosis Using Longitudinal EEG Data. Journal of Neural Engineering, 2019, 16(5): 056031. doi: 10.1088/1741-2552/ab22ea. |
[18] |
Vansteensel MJ, Pels EGM, Bleichner MG, et al. Fully Implanted Brain-Computer Interface in a Locked-In Patient with ALS. New England Journal of Medicine , 2016, 375(21): 2060-2066. doi: 10.1056/NEJMoa1608085. |
[19] |
Donchin E, Spencer KM, Wijesinghe R. The Mental Prosthesis: Assessing the Speed of a P300-based Brain-computer Interface. IEEE Transactions on Rehabilitation Engineering, 2000, 8(2): 174-179. DOI: 10.1109/86.847808. |
[20] |
Aricò P, Borghini G, Di Flumeri G, et al. Adaptive Automation Triggered by EEG-Based Mental Workload Index: a Passive Brain-Computer Interface Application in Realistic Air Traffic Control Environment. Frontiers in Human Neuroscience, 2016, (10): 539. |
[21] |
Wei CS, Wang YT, Lin CT, et al. Toward Drowsiness Detection Using Non-hair-Bearing EEG-Based Brain-Computer Interfaces. IEEE Transactions on Neural Systems and Rehabilitation Engineering, 2018, 26(2): 400-406. doi: 10.1109/TNSRE.2018.2790359. |
[22] |
Wu D, Lance BJ, Lawhern VJ, et al. EEG-Based User Reaction Time Estimation Using Riemannian Geometry Features. IEEE Transactions on Neural Systems and Rehabilitation Engineering, 2017, 25(11): 2157-2168. doi: 10.1109/TNSRE.2017.2699784. |
[23] |
Vourvopoulos A, Pardo OM, Lefebvre S, et al. Effects of a Brain-Computer Interface With Virtual Reality (VR) Neurofeedback: a Pilot Study in Chronic Stroke Patients. Frontiers in Human Neuroscience, 2019, (13): 460405. |
[24] |
Horki P, Solis-Escalante T, Neuper C, et al. Combined Motor Imagery and SSVEP Based BCI Control of a 2 DoF Artificial Upper Limb. Medical & Biological Engineering & Computing, 2011, 49(5): 567-577. |
[25] |
Singh AK, Wang YK, King JT, et al. Extended Interaction With a BCI Video Game Changes Resting-State Brain Activity. IEEE Transactions on Cognitive and Developmental Systems, 2020, 12(4): 809-823. DOI: 10.1109/TCDS.2020.2985102. |
[26] |
Choi B, Jo S. A Low-Cost EEG System-Based Hybrid Brain-Computer Interface for Humanoid Robot Navigation and Recognition. PLOS ONE, 2013, 8(9): e74583. DOI: 10.1371/journal.pone.0074583. |
[27] |
|
[28] |
Brunner C, Birbaumer N, Blankertz B, et al. BNCI Horizon 2020: towards a Roadmap for the BCI Community. Brain-Computer Interfaces, 2015, 2(1): 1-10. doi. 10.1080/2326263x.2015.1008956. |
[29] |
Min BK, Marzelli MJ, Yoo SS. Neuroimaging-based Approaches in the Brain-computer Interface. Trends in Biotechnology, 2010, 28(11): 552-560. doi. 10.1016/j.tibtech.2010.08.002. |
[30] |
Rosenfeld JV, Wong YT. Neurobionics and the Brain-computer Interface: Current Applications and Future Horizons. Medical Journal of Australia, 2017,. 206(8): 363-368. doi: 10.5694/mja16.01011. |
[31] |
Matthews F, Pearlmutter BA, Wards TE, et al. Hemodynamics for Brain-Computer Interfaces. IEEE Signal Processing Magazine, 2008, 25(1): 87-94. DOI. 10.1109/msp.2008.4408445. |
[32] |
Fukuma R, Yanagisawa T, Saitoh Y, et al. Real-Time Control of a Neuroprosthetic Hand by Magnetoencephalographic Signals from Paralysed Patients. Scientific Reports, 2016, 6(1): 21781. doi: 10.1038/srep21781. |
[33] |
Kaas A, Goebel R, Valente G, et al. Topographic Somatosensory Imagery for Real-Time fMRI Brain-Computer Interfacing. frontiers in Human Neuroscience. 2019, (13): 427. |
[34] |
Faress A, Chau T. Towards a Multimodal Brain-computer Interface: Combining fNIRS and fTCD Measurements to Enable Higher Classification Accuracy. NeuroImage, 2013, (77): 186-194. |
[35] |
Lu J, Mamun KA, Chau T. Pattern Classification to Optimize the Performance of Transcranial Doppler Ultrasonography-based Brain Machine Interface. Pattern Recognition Letters, 2015, (66): 135-143. |
[36] |
Khalaf A, Sejdic E, Akcakaya M. A Novel Motor Imagery Hybrid Brain Computer Interface Using EEG and Functional Transcranial Doppler Ultrasound. journal of Neuroscience Methods, 2019, (313): 44-53. |
[37] |
Pandarinath C, Nuyujukian P, Blabe CH, et al. High Performance Communication by People with Paralysis Using an Intracortical Brain-computer Interface . Elife, 2017, (6): e18554. |
[38] |
Kaiju T, Doi K, Yokota M, et al. High Spatiotemporal Resolution ECoG Recording of Somatosensory Evoked Potentials with Flexible Micro-Electrode Arrays . Frontiers in Neural Circuits, 2017, (11): 20. |
[39] |
Lajoie G, Krouchev NI, Kalaska JF, et al. Correlation-based Model of Artificially Induced Plasticity in Motor Cortex by a Bidirectional Brain-computer Interface. plos Computational Biology, 2017, 13(2): e1005343. doi: 10.1371/journal.pcbi.1005343. |
[40] |
Darvishi S, Gharabaghi A, Boulay CB, et al. Proprioceptive Feedback Facilitates Motor Imagery-Related Operant Learning of Sensorimotor β-Band Modulation. frontiers in Neuroscience, Frontiers, 2017, (11): 60. |
[41] |
Bhattacharyya S, Clerc M, Hayashibe M. Augmenting Motor Imagery Learning for Brain-Computer Interfacing Using Electrical Stimulation as Feedback. IEEE Transactions on Medical Robotics and Bionics, 2019, 1(4): 247-255. doi: 10.1109/TMRB.2019.2949854. |
[42] |
Bockbrader M, Annetta N, Friedenberg D, et al. Clinically Significant Gains in Skillful Grasp Coordination by an Individual with Tetraplegia Using an Implanted Brain-Computer Interface with Forearm Transcutaneous Muscle Stimulation. Archives of Physical Medicine and Rehabilitation, 2019, 100(7) : 1201-1217. doi: 10.1016/j.apmr.2018.07.445. |
[43] |
Murovec N, Heilinger A, Xu R, et al. Effects of a Vibro-Tactile P300 Based Brain-Computer Interface on the Coma Recovery Scale-Revised in Patients With Disorders of Consciousness. Frontiers in Neuroscience, 2020, (14): 294. |
[44] |
Gonçalves SI, de Munck JC, Pouwels PJW, et al. Correlating the Alpha Rhythm to BOLD Using Simultaneous EEG/fMRI: Inter-subject Variability. NeuroImage , 2006, 30(1): 203-213. doi: 10.1016/j.neuroimage.2005.09.062. |
[45] |
Käthner I, Wriessnegger SC, Müller-Putz GR, et al. Effects of Mental Workload and Fatigue on the P300, Alpha and Theta Band Power during Operation of an ERP (P300) Brain-computer Interface. Biological Psychology, 2014, (102): 118-129. |
[46] |
Calhoun VD, Adali T. Time-Varying Brain Connectivity in fMRI Data: Whole-brain Data-driven Approaches for Capturing and Characterizing Dynamic States. IEEE Signal Processing Magazine, 2016, 33(3): 52-66. DOI: 10.1109/MSP.2015.2478915. |
[47] |
Bashashati A, Fatourechi M, Ward RK, et al. A Survey of Signal Processing Algorithms in Brain-computer Interfaces Based on Electrical Brain Signals. Journal of Neural Engineering, 2007, 4(2): R32. DOI: 10.1088/1741-2560/4/2/R03. |
[48] |
Pfurtscheller G, Neuper C. Motor Imagery and Direct Brain-computer Communication. Proceedings of the IEEE, 2001, 89(7): 1123-1134. DOI: 10.1109/ 5.939829. |
[49] |
Lawhern VJ, Solon AJ, Waytowich NR, et al. EEGNet: A Compact Convolutional Neural Network for EEG-based Brain-computer Interfaces. journal of Neural Engineering, 2018, 15(5): 056013. doi: 10.1088/1741-2552/aace8c. |
[50] |
Schirrmeister RT, Springenberg JT, Fiederer LDJ, et al. Deep Learning with Convolutional Neural Networks for EEG Decoding and Visualization. Human Brain Mapping, 2017, 38(11): 5391-5420. doi: 10.1002/hbm.23730. |
[51] |
Kasahara K, DaSalla CS, Honda M, et al. Neuroanatomical Correlates of Brain-computer Interface Performance. NeuroImage, 2015, (110): 95-100. |
[52] |
Lotte F, Congedo M, Lécuyer A, et al. A Review of Classification Algorithms for EEG-based Brain-computer Interfaces. journal of Neural Engineering,. 2007, 4(2): R1-R13. DOI: 10.1088/1741-2560/4/2/R01. |
[53] |
Mane SAM, Shinde A. StressNet: Hybrid Model of LSTM and CNN for Stress Detection from Electroencephalogram Signal (EEG). Results in Control and Optimization, 2023, (11): 100231. |
[54] |
Cecotti H, Graser A. Convolutional Neural Networks for P300 Detection with Application to Brain-Computer Interfaces. IEEE Transactions on Pattern Analysis and Machine Intelligence, 2010, 33(3): 433-445. |
[55] |
Craik A, He Y, Contreras-Vidal JL. Deep Learning for Electroencephalogram (EEG) Classification Tasks: a Review. Journal of Neural Engineering, 2019, 16(3): 031001. doi: 10.1088/1741-2552/ab0ab5. |
[56] |
Kleih SC, Kübler A. Empathy, Motivation, and P300 BCI Performance. Frontiers in Human Neuroscience, 2013, (7): 642. |
[57] |
Rajesh P. N. Rao. Introduction to Brain-Computer Interfaces. Translated by L. Zhang, M. U. Chen. Beijing: Machinery Industry Press, 2016. |
[58] |
Kaufmann T, Vögele C, Sütterlin S, et al. Effects of Resting Heart Rate Variability on Performance in the P300 Brain-computer Interface. International Journal of Psychophysiology, 2012, 83(3): 336-341. DOI: 10.1016/j.ijpsycho.2011.11.018. |
[59] |
Mantini D, Perrucci MG, Del Gratta C, et al. Electrophysiological Signatures of Resting State Networks in the Human Brain. Proceedings of the National Academy of Sciences, 2007, 104(32): 13170-13175. DOI: 10.1073/pnas.0700668104. |
[60] |
Vidaurre C, Blankertz B. Towards a Cure for BCI Illiteracy. Brain Topography, 2010, 23(2): 194-198. DOI: 10.1007/s10548-009-0121-6. |
[61] |
Sporns O. Structure and Function of Complex Brain Networks. Dialogues in Clinical Neuroscience, 2013, 15(3): 247-262. DOI: 10.31887/DCNS.2013.15.3/ osporns. |
[62] |
WU Zhaohui, YU Yipeng, PAN Gang, et al. A review of brain-computer fusion systems. Life Sciences, 2014, 26(6): 645-649. |
[63] |
Shaeri M, Shin U, Yadav A, et al. A 2.46-mm ^2 Miniaturized Brain-Machine Interface (MiBMI) Enabling 31-Class Brain-to-Text Decoding. https:// ieeexplore.ieee.org/document/10643873.[2024-10-1]. |
[64] |
Benchetrit Y, Banville H, King JR. Brain Decoding: toward Real-time Reconstruction of Visual Perception. https://scholar.ucas.ac.cn/https/ 77726476706e69737468656265737421f1e55995317e674279/abs/2310.19812.[2024-10-1]. |
[65] |
TAO Hu, SUN Lui-Yang. Realization of "human-machine-object" ternary fusion--Talking about the advantage of human brain in brain-computer interface. Frontier Science, 2021, 15(2): 104-108. DOI: 10.3969/j.issn.1673-8128.2021.02.024. |
[66] |
Yang D, Nguyen TH, Chung WY. A Bipolar-Channel Hybrid Brain-Computer Interface System for Home Automation Control Utilizing Steady-State Visually Evoked Potential and Eye-Blink Signals. Sensors, 2020, 20(19): 5474. DOI: 10.3390/s20195474. |
[67] |
Wu D, King JT, Chuang CH, et al. Spatial Filtering for EEG-Based Regression Problems in Brain-Computer Interface (BCI). IEEE Transactions on Fuzzy Systems, 2018, 26(2): 771-781. doi: 10.1109/TFUZZ.2017.2688423. |
[68] |
Coogan CG, He B. Brain-Computer Interface Control in a Virtual Reality Environment and Applications for the Internet of Things. IEEE Access, 2018, (6): 10840-10849. |
[69] |
Zhang X, Yao L, Zhang S, et al. Internet of Things Meets Brain-Computer Interface: a Unified Deep Learning Framework for Enabling Human-Thing Cognitive Interactivity. IEEE Internet of Things Journal, 2019, 6(2): 2084-2092. doi: 10.1109/JIOT.2018.2877786. |
[70] |
Chu NNY. Surprising Prevalence of Electroencephalogram Brain-Computer Interface to Internet of Things [Future Directions]. IEEE Consumer Electronics Magazine, 2017, 6(2): 31-39. doi: 10.1109/MCE.2016.2640599. |
[71] |
Aloise F, Schettini F, Aricò P, et al. P300-based Brain-computer Interface for Environmental Control: An Asynchronous Approach. Journal of Neural Engineering, 2011, 8(2): 025025. DOI: 10.1088/1741-2560/8/2/025025. |
[72] |
Ruan Meihua, Zhang Liwen, Ling Jiefan, et al. Development of brain-computer interface field in 2023. Life Sciences, 2024, 36(1): 39-47. |
[73] |
Wang J, Wang C, Cai P, et al. Artificial Sense Technology: Emulating and Extending Biological Senses. ACS Nano, 2021, 15(12): 18671-18678. DOI: 10.1021/ acsnano.1c10313. |
[74] |
Berger TW, Hampson RE, Song D, et al. A Cortical Neural Prosthesis for Restoring and Enhancing Memory. Journal of Neural Engineering, 2011, 8(4): 046017. DOI: 10.1088/1741-2560/8/4/046017. |
[75] |
Zhang Zhe, Zhao X, Ma Yixin, et al. Consideration of ethical codes for brain-computer interface technology. Journal of Biomedical Engineering, 2023, 40(2): 358-364. DOI: 10.7507/1001-5515.202208058. |
[76] |
Wang Gaofeng, Zhang Zhilun. A study on the ethical issues of brain-computer interfaces under the perspective of algorithmic ethics. Natural Dialectics Research, 2022, 38(7): 68-73. |
Brain-Computer Interface Technology: Current development status and future outlook
Xiao Song is an associate researcher of Hubei Science and Technology Information Research Institute. His research interests include science and technology management, science and technology policy and strategic planning.
Cheng Heping is a professor at Peking University and an academician of the Chinese Academy of Sciences. His research interests include calcium signaling, mitochondrial function research, and creation of research instruments.
Zhaohui Wu is a professor at Zhejiang University and an academician of the Chinese Academy of Sciences. His research interests include artificial intelligence, brain-computer intelligence, and service computing.
Xu Zhang is a researcher at Guangdong Institute of Intelligent Science and Technology. His research interests include neuroscience, molecular cell biology mechanisms of neurological diseases.
Wang Yizheng is a researcher at the Institute of Military Medical Sciences, Academy of Military Sciences. His research interests include brain diseases and brain-like research.
Jing Chen is a researcher at Peking University. Her research interests include speech information processing, machine hearing, and speech neural decoding.
Pan Gang is a professor at Zhejiang University. His research interests include artificial intelligence, brain-computer interface, and brain-like computing.
Tao Hu is a researcher at Shanghai Institute of Microsystems and Information Technology, Chinese Academy of Sciences. His research interests include brain-computer interface, artificial intelligence, and micro-nano opto-mechanical systems.
Yao Dezhong is a professor at the University of Electronic Science and Technology. His research interests include neural information and brain-computer interaction.
Xiaojie Duan is a long term associate professor at Peking University. His research interests are in biomedical engineering.
Gang Wang is an associate researcher at the Institute of Military Medical Research, Academy of Military Science. His research interests are brain-like visual computing.
Corresponding author:
Cheng Heping at chengp@pku.edu.cn.
Project Grants:
Chinese Academy of Sciences (CAS), "Brain-computer interface key scientific issues, key core technologies and their layout" (2023-ZW07-A-026).
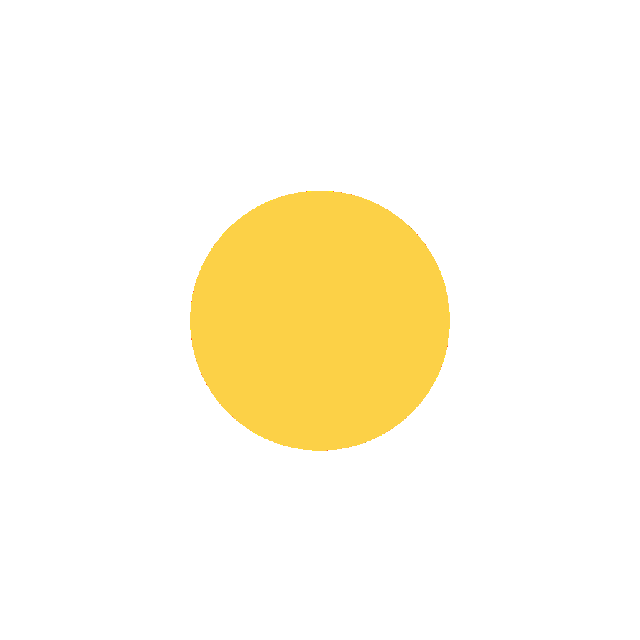
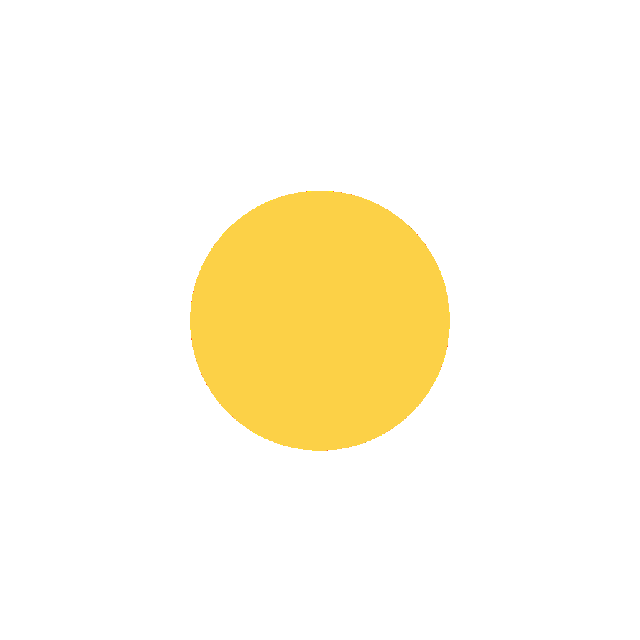
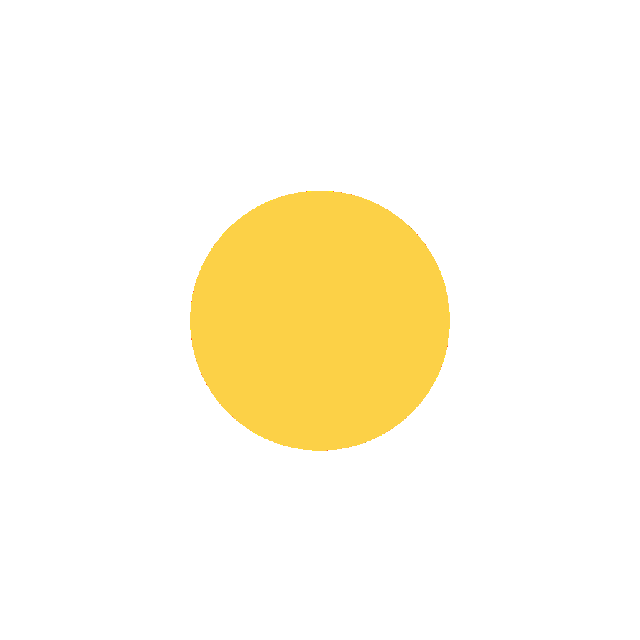
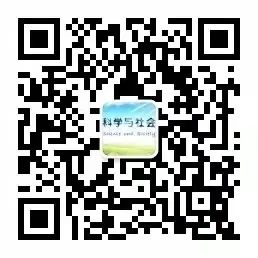
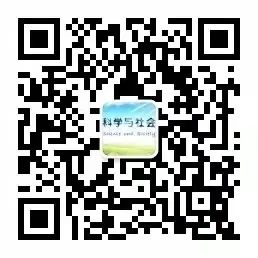
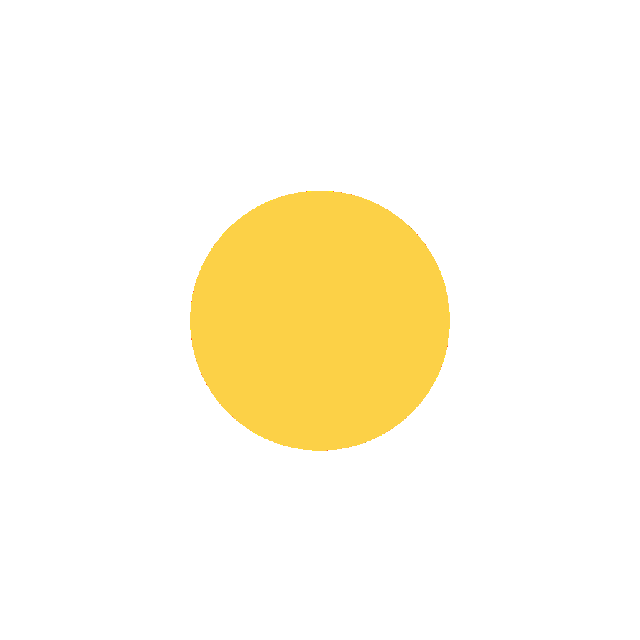
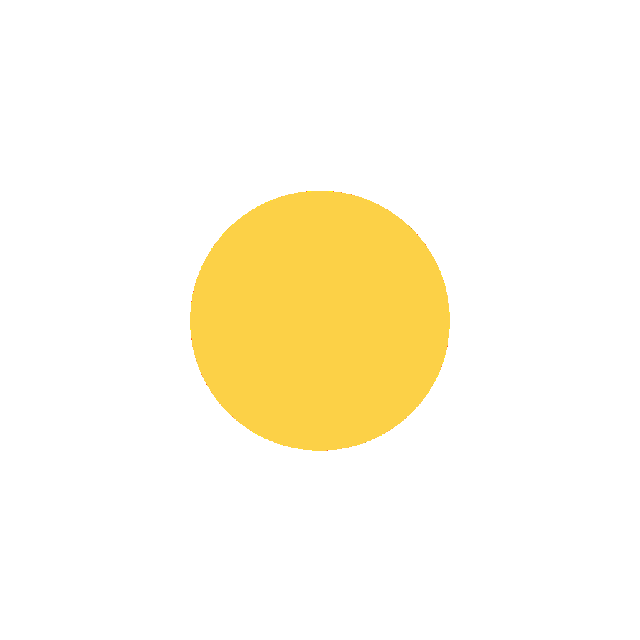
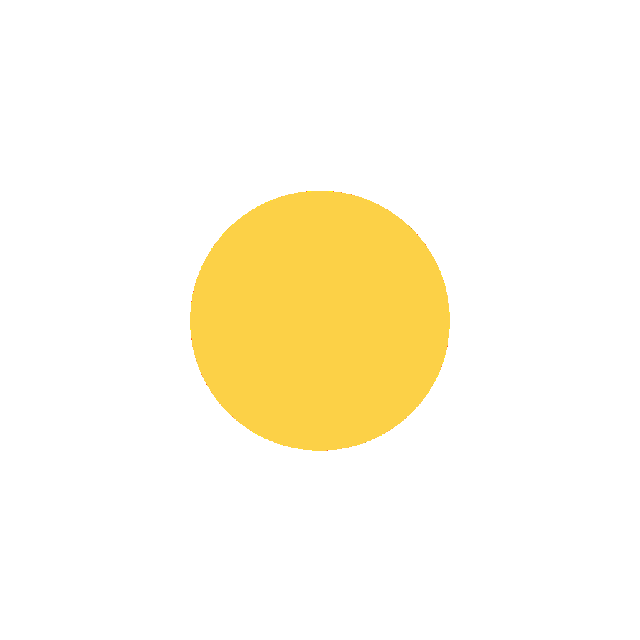
Science and society
Interaction between science and society
Cultural heritage of science and tradition
Science and humanities meet and collide
Free Discussion of Science and Ideas